Dirty Data: How Democratizing Access to Data is Failing to Improve Organizational Decision Making
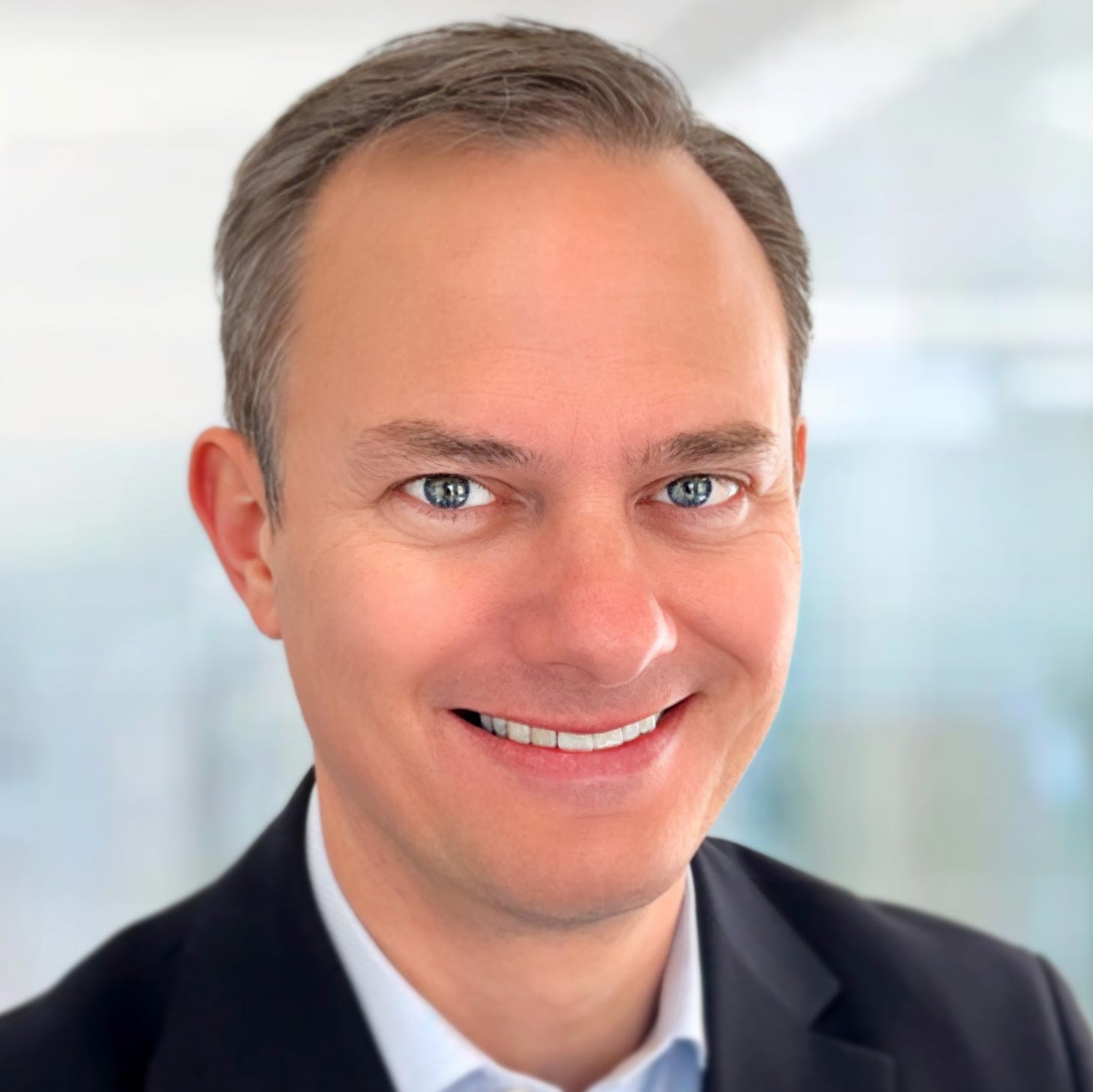
It’s a well-known fact that many organizations struggle to make data-driven decisions. And there’s also a widely-accepted hypothesis that lack of access to data is the reason why it’s so difficult. After all, if organizations could simply make it easier for decision makers to access the right data at the right time, then decision making would improve.
Over the past decade or more, companies have spent a lot of time and energy democratizing data. Said differently, companies have provided everyone, regardless of their technical know-how, a self-service way to easily work with the data, understand the data, and confidently use it to make data-driven decisions. It’s why products like Qlik, Tableau, and PowerBI exist. The thinking? If decision makers could easily access data and build their own charts and graphs with it to answer business questions, then they would make better, data-driven decisions.
Democratizing data is certainly a laudable goal. In fact, for many companies, this is where they are on their journey to become data-driven. And for the most part, we’ve solved this challenge and provided great tools that give companies access to all the data they need. But now, we’re realizing that we actually have another problem on our hands: the data behind the visualizations and dashboards is junk. This problem isn’t new. But while we’ve fixed the veneer with tools that make it easy to access and manipulate data, we’ve now exposed data’s rotten core.
What is Dirty Data – and Why Does it Exist?
Junky or dirty data is data that is incorrect, incomplete, inconsistent, out-of-date, duplicative – or all of the above. It’s a common issue because in many companies, data is trapped in silos across the organization. These silos represent the organization of the company: Marketing, Product, Sales, Operations, or R&D teams. In software development, we call this Conway’s Law, an engineering principle that says the software products a company develops reflect the structure of the organization that wrote them. As a side note, do I get to coin the term Deighton’s Law: data reflects the organizational and systems structure of the company that generates it?
In data terms, that means that the source systems reflect the structure and organization of the business. Here’s a dirty data example: if a telecommunications company has five lines of business (LOB), then they likely have five (or more!) different source systems, each supporting a specific LOB. So when a decision maker creates a dashboard in their self-service analytics tool, they create a dashboard for a single LOB because that is what the data source reflects.
But even within these departmental systems, the data is dirty. That’s reality. And that’s also why it is difficult for organizations to reconcile their data across systems and silos. We experience the result of this junky, inconsistent data as consumers. Perhaps you have multiple services such as internet and television with a single provider, but each service is under a slightly different name or address. The provider is unable to recognize that you are the same person, and therefore they communicate with you multiple times as though you were totally separate individuals.
To complicate matters even further, the best version of the data is unlikely to exist within your company. Said differently, to improve the quality of your data, you need to look outside your firewall. Think about it. If you are a manufacturer looking for the cleanest data about your supplier, it’s unlikely that the data you pull from your enterprise resource planning (ERP) system is the most accurate. Instead, the best copy of this known entity would come from the supplier’s website or Dun & Bradstreet’s database as an example.
So even though we’ve solved the access and democratization issue, we have not yet solved the problem that the underlying data is dirty and messy. And that’s why so many business leaders are making bad decisions.
To be fair, we really haven’t given users a good way to solve the issue. Many companies, self-service analytics vendors included, have attempted to solve the dirty data problem through band aid approaches focused on the front-end data load. Others combine data sources manually through the ever-popular “export to Excel” feature. In this example, users take data from multiple sources, mash it together in Excel, and rationalize it manually using a series of Excel formulas and vlookups.
Still others believe that a single solution, used across the entire organization, will solve the dirty data problem once and for all. They’ll implement a single ERP or a single CRM or a consolidated data warehouse with the hope that having all the data consolidated in one place will keep it clean. But unfortunately, despite the best of intentions, there will always be other data in other systems and sources.
I believe that it’s time for a different, arguably better, approach.
A New – and Better – Approach to Cleaning Dirty Data
To truly fix bad, junky data, we believe you need another layer. One that sits between your front-end data preparation tools and your messy back-end source systems and resolves the business entities that matter to your company. This business context layer allows you to transform the data into business topic areas that matter to your decision makers. And it’s this layer that provides a business-oriented lens that provides visibility into the business topic areas that matter to your business such as:
- Who are my customers or suppliers?
- What parts do we use?
- Who are the people we work with?
- What products do we ship?
If you believe, as I do, that creating this lens is useful, then you must embrace machine learning. Attempting to create this view manually is a fool’s errand, as there is simply too much bad source system data and it changes too frequently to find the meaning and show it to your users. Machine learning gives your organization the power to make these views possible.
Tamr’s cloud-native, machine learning-driven data mastering enables you to fix dirty data at the point of consumption. Using machine learning, you can create a view into your messy data that provides a business-oriented lens, enabling decision-makers to gain visibility into the business topic areas they care about the most. Then, they can use these business topic areas to power analytics for better, more informed decision making.
Dirty data, unfortunately, is not going away. But adding a new layer between your messy, ever-changing source system data and your shiny new visualization front-end tools will enable you to translate data into the entities that matter most to your business. And this new lens will help your decision makers make better, more confident decisions because the data that powers their self-service analytics will be oriented to the business topic areas that matter most to your organization.
To learn more, schedule a demo.
Get a free, no-obligation 30-minute demo of Tamr.
Discover how our AI-native MDM solution can help you master your data with ease!