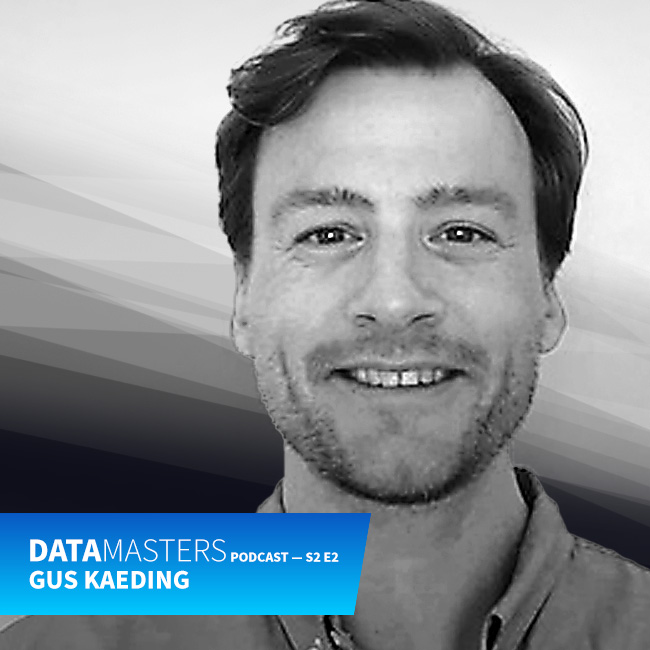
U.S. Ski & Snowboard Team's Gus Kaeding: Mining data for gold medals
Gus Kaeding
Gus Kaeding talks to Anthony about how the U.S. Ski & Snowboard teams are using data to improve team performance and win more medals. Gus explains where the similarities start and end with the famous "Moneyball' approach to team selection. Why communication with coaches and athletes about data findings is just as important as the results. The challenges of starting a data program from scratch, and what's next for the team as they approach the 2020 Winter Olympics.
I'd rather read the transcript of this conversation please!
Anthony: Welcome back to season two, episode two of DataMasters. I'm really excited about our guest this week, Gus Kaeding. So far, all of our guests have been from the business world, academia or the government. However, Gus is unique. He's applying his data know-how and expertise to a different arena. The arena of athletics, he's the performance data manager for the US Ski and Snowboard Team, a role in which he oversees data and analytics for seven sports, 17 teams, and over a hundred athletes. Gus's work is pivotal in the lead up in preparation for the 2022 Winter Olympics in Beijing. Welcome Gus and thanks for coming on DataMasters.
Gus Kaeding: Hi Anthony, thanks for having me, excited to be here.
Anthony: Awesome. So I thought we could start our conversation more at a personal level and talk a little bit about your personal history and I think it's fair to say in the world of data and analytics, you may have the most coveted job of all working with skiers. Presumably you do all your analytics on the ski slope. Now in seriousness, I'm curious, how do you become the performance data manager for the US Ski and Snowboard Team?
Gus Kaeding: Yeah, well, it was a long time in the making and a lot of self discovery along the way, but I started in as an athlete and in coaching with the US Ski Team and I had a mathematics background from college and I had always wanted to apply that to something else going forward. So when I saw the end of the coaching realm for me, I decided to go back to school and I got my MBA when I did that. I wrote my essays on starting a new department at US Ski and Snowboard that was an analytics department. And going to school in Boston, there's all sorts of awesome opportunities there. The MIT Sports Analytics Conference, there's just a lot of data being used in whatever industry.
Gus Kaeding: And so I was able to return to US Ski and Snowboard in a different role following graduate school and a role that had not existed to this point. And I was actually hired into that role by pitching a process to the US Ski Team about using data for team selection and how data could be used to give our team a competitive advantage over other Olympic teams going forward.
Anthony: So you come at the role from the perspective of a practitioner and as an athlete, do you think that gives you a sense of empathy and maybe reality for the impact that data and analytics can have for a skier?
Gus Kaeding: Yeah, I think in probably any industry, but where I've seen it most clearly used in sports, the collision, I would say between athletics with high emotions and a lot of just gut feelings and cold hard data is a tough one. And having a background, not only as a coach, but also as an athlete has given me not only a little bit more insight of how to best communicate some of those insights and challenges, but also just to get buy-in from all of the stakeholders, whether they're athletes or coaches and what they're really trying to solve for, and allowing me to help as part of that process, as opposed to just delivering demands and it's really more of a community effort as opposed to two sides working against each other.
Anthony: Yeah. I think this is probably a theme many of our listeners are quite familiar with and this idea of creating alignment between a data function, the business function in here, you're trying to do it between a data function and sports and performance and athletes. And as you point out like gut and demotion and the way things always have been are often strong drivers for decision-making in the sports arena. I think, one of the things that we're sure is on everyone who's listening mind is the book and movie Moneyball, which talked about how data and analytics fundamentally [inaudible 00:05:57] in that case Major League Baseball, is there an element of Moneyball for skiing that's going on here? Is that a fair analogy or an unfair analogy?
Gus Kaeding: Yeah. It's funny you bring that up. When I first started this position, I told my mom when I'd be doing, and she was like, "Are you going to be like a Brad Pitt?" And I was like, well, I think it more is the other guy. And that was the last time she asked me about my job in the last three years.
Anthony: I love it.
Gus Kaeding: But actually in business school, in one of our strategy classes, they played that clip with Brad Pitt and all the Scouts, what is the problem? What is the problem? Over and over and over again. And that is exactly where we are at with the US Ski Team. And we are trying to identify the problem. And the difference between us and baseball is when Brad Pitt identifies the problem, it's players who get on base percentage.
Gus Kaeding: And essentially our problem is getting athletes from the start line to the finish line, as fast as possible, or from the start line to the finish line, with as high a score as possible in some of our judge sports. The key difference and I think the really fun part is baseball had these troves of data that they had collected over the years for baseball cards and whatever. We have no data, we had no data. And so we got a fresh start at all of these problems.
Gus Kaeding: And the tricky part is when you identify a problem and you're working with no data, you're essentially identifying a problem that you want to address three years down the road. So you have to think way ahead and your strategies have to be really aligned across all departments. So we've tried to do that when I was hired. And now it's year three for me, going to year four. And it's the exciting time because I feel we've gotten enough data where we can begin to really answer some of those questions that we've sought out that we established from the start. So switching from collection to analysis now, which is super exciting.
Anthony: Yeah. Again, a theme. I think I'm sure many listeners resonate with this idea that you go to answer a business question and the responses were we don't have that data or that data is trapped in five different silos and the first order of business is to bring it together. I'm sensitive to the fact that, as you point out, like you've spent a lot of time collecting this data in many cases, not collecting in the sense of finding and bringing it together, but collecting it in the sense of actually measuring it for the first time. One of the fun parts about Moneyball as a story, and you pointed out on base percentage is this key metric, but the key metric also resulted in some counter-intuitive conclusions. The team that there is put together, in a very literal sense didn't look like a conventional baseball team but was outfitted appropriately across the metrics that matter. Have you found any similar counter-intuitive conclusions from a skiing perspective or are there counterintuitive hypotheses that you're working to validate?
Gus Kaeding: You know, probably the one that jumps out earliest on and is still the most controversial is the funding of our programs. So in business school, I did strategy and finance. And so, one of the things I've tried to align myself here with is linking data to more resource allocated decisions. And right from the start we name our teams... Well, before I arrived, we named our teams generally just off of gut, off of discretion, off of what the coaches saw. And is there a place for that? Absolutely. But should you be adding in a data perspective to have more informed decisions? Yes. And so when I did an analysis of the way we had been naming teams, I identified that there was a group of athletes at the higher level who really didn't fall into the goal of the organization, which is winning medals.
Gus Kaeding: And so the question became, does an athlete who has a lot of experience is a little older and has progressed to here, does he have or he, or she have a better chance of winning a medal as a younger than a younger athlete? And that was something we were able to look at a lot of historical data on and really break it down just by simple percentages and look at percentage chance to win a medal at any point in your career.
Gus Kaeding: And the analysis was done and we ended up really building out a more robust development system. So depending on the sport, a lot more, 15, 16, 17, 18 year old athletes, and a couple of sports that have really grabbed on to that and really built out that system are really starting to see success now. And I had anticipated it would land in the 2026 Olympics, but we really might see a couple of really young Olympians from from the US side here in 2022. And that's really exciting. So it's basically just a re allocation of resources, whether it's coaching, money, focus. And that was one of our key early insights.
Anthony: I love that example because I think it's very analogous to what we see in business. You might have an emerging product line and the tendency is to allocate resources consistent with how you've done it in prior years, which is very analogous to what you talked about, which is allocating resources to the skiers who've shown a commitment to the program. Who've been there for some time. And that's like the analogy of putting resources into the products that are dominant in the market. But what you point out is that to achieve the goal and you frame the goal so well to win medals and in business that could be share price or market share, or revenue performance, profitability. To achieve the goal, you might need to make some bets on the young high-potential and in your case, young high-potential skiers, but in business could be the young, high potential business units or product lines. So what do you think, is that fair?
Gus Kaeding: Yeah, I think that's absolutely fair. I would say that the young potential would be like the R and D department thinking a little bit more long-term. The difficult aspect certainly is the communication of these changes. And using your example, it would be like phasing out a favorite line of some product that's getting a little long in the tooth and bringing in something that maybe you expect to have more success in the future. And that is a difficult conversation to have. The one thing that we really have behind us is we are able to frame up that goal and then support our decisions with data. And then the previous way, the argument is just, that's how we've always done it. And I think very well understood across the business world now and increasingly well across the sports world is that is not a good excuse anymore. And watch out for when that jumps into your mind when approaching a problem.
Anthony: And did you find that using data, it's a way of insulating the personal in that conversation? I mean, for a skier and in a sports program, it's truly personal, you're talking about human beings, at least in the business world, we're talking about a product line and hopefully product product lines don't have feelings, but does it help to turn to data?
Gus Kaeding: Yeah, I think it does. And I'm not sure it helps to turn to data in hindsight, but one of the challenges even before was athletes had no expectation or clarification of why they would have been on or off the team. It was really just up to the coaches. So it's that ambiguity that really leads to those tough solutions and tough things in the athletes and community mind. But when you're able to lay out beforehand the data points, the one, two, three, this is [inaudible 00:15:57], what's going to take, and if you achieve any of these, you're on the team, no ifs, ands, or buts, then they get to the end of the season, they can say, "Okay, did I achieve this or not? No, I did not." And that is a lot clearer in their mind and can really help with that conversation, following that, make it a little easier.
Anthony: I love it. So that's a great lesson for everyone listening to take is helping the... Having the data help and also having it be something that you talk about ahead of making some of these difficult decisions-
Gus Kaeding: Managing expectations, a really important part of all this, I feel.
Anthony: So the other challenge with athletes, we've talked a lot about how the coaches and the team are using data to rethink how they select who's on the team. But I know that one of the places you've been investing a lot of energy is in actually sharing the data with the athlete themselves and changing individual behavior. Do you want to share a little bit about how that works and how you're using data with individual skiers?
Gus Kaeding: Yeah. I think anytime you're delivering data, you have to think really carefully about your method of delivery. And I listened to your previous podcasts with Kathleen Malley, which was exceptional, and I thought she framed up this problem really well. And so for me, I'm essentially two steps removed from the athletes, but I'm trying to affect athlete performance. So my decision is, do I give this insights or direct data to the coaches or do I give it to the athletes? And generally to keep one of my key stakeholders happy, I do give it directly to the coaches and I allow them to filter it to the athletes as they see fit. Now, every once in a while, I get the privilege of going directly to the athlete and oftentimes, every time I will include the coach in that conversation, you have to keep everyone in the room happy. That's step one.
Gus Kaeding: But a fun example recently that we've done is I think we have a world-class medical department and they had the foresight a number of years ago to start really thoughtfully collecting ACL injury data. And that is one of our biggest injuries each year at any given time on any given team, we can have up to 40% of our athletes out, which is just really a hindrance, especially approaching a big competition. So over the years, we've been able to develop a pretty good dataset of ACL injuries. And recently went through an analysis of what went into each ACL injury, whether it was different snow type, different mechanism of injury, then different surgery type, different graph type, different surgeon, different location, rehab location, rehab length, different markers throughout the rehab. And one of the exciting projects we were just able to wrap up was looking at what factors led to those ACL injuries.
Gus Kaeding: And that's something that the athletes really care about. They might not be so apt to hear that I'm on this team, I'm not on this team. But when you're telling them these are one, two, and three things you can do to help keep you healthy. That is ultimately beneficial to them, will help keep them on the course. Will help keep those sponsors interested, more money for them, and will help buy in with our own programs. And ultimately, maybe fill out that next survey that I send, or when the next project comes around, those are the key stakeholders that I want to engage and to keep happy. So that's just one example of how we're trying to work with the athletes and the coaches directly.
Anthony: That's great. And the link to think about there is, the coach is a little bit like the manager and the athlete is like person, the individual contributor, this idea of keeping all the stakeholders involved in the communication. You don't just drop a bunch of data on the coach and expect them to be able to communicate it clearly to the skier. And you don't also, just have the skier taking a look at data and expect them to be able to dispassionately draw conclusions. For them, it's a passion. It's their job. And especially in the context of injuries that passion can often have, I assume, have skiers making decisions of wanting to get back on the course too quickly to push themselves. Have you been able to share insights with them about that?
Gus Kaeding: Yes, but I would say with an asterisk, it's the hottest topic in sports these days, if you look across any of the pro sports, looking at injury prevention is one of the biggest trends. And so one of the ways we do it is athletes they train every day and they record their training. And what we look at with that training is their load. And so basically how hard was a given day on the athlete's body. And one of the metrics we use in this is called RPE and it really is a load measurement day by day. And there's very good data that shows if an athlete's chronic load becomes lower than their acute load. Meaning if they've worked too hard recently with no base before that they are more susceptible to injury.
Gus Kaeding: So what we do is every day when they enter their training, basically have this algorithm updating and right on their phone or dashboard or wherever they choose to have it, they can see what percentage they are at in this acute to chronic ratio. And they know that if they get above a certain threshold, I got to back it off for a few days because I am now opening myself up to injury and injury prevention is one of the biggest things that we look at because you can't improve unless you are out there training and racing on a daily basis.
Anthony: Yeah. And you can't win a medal with an ACL. So you've really got to keep these athletes injury-free if you're going to achieve the ultimate goal of the organization, which is more medals. So I think that's a great example. I love that idea of operationalizing that metric. This idea that the athlete is every day looking at that number and using it as a way to remove their emotional reaction to I've just got to push harder for one more day. And and that can be the difference between being on the slope in Beijing and not.
Gus Kaeding: Yeah. The interesting concept there that... Whether it's that ratio or any other metric is you spoke to the different managers and their different styles, each athlete and each coach is different too. And some coaches you give them a metric and they're going to be on that metric every day, no matter what. And that's just not how data works. There's gray areas and there's other considerations. And so that's the fun part to me is managing the different personalities and knowing how you have to deliver these messages a little differently. Some coaches, you just send them your coding script and that's all they want. But some of the other ones you have to sit down with a beer and explain this is why we're doing this, and this is the metric. But if you vary a little bit here or there that's okay, but give a call and we'll discuss it through. So really interesting to work with the different personalities and different understandings of data throughout this.
Anthony: Yeah. So this idea that ultimately as in business, the people doing the work are people. So they have to engage with the data in a very human way. It's filtered through, as you point out their personality, maybe even their capability, some may understand things more intuitively have been more data-driven in their training, others less so. And so you need to meet them where they are.
Gus Kaeding: Yeah, exactly. And I actually think it's important to use them as a sense check as well. Lots of times you're delivering insights that may go against a bias, but when they raise a red flag, that's something I want to take back and look at, because it's actually quite interesting when you look at these insights and you deliver them to them. Most of the time they say, yeah that looks about right. And so I think the human mind generally is quite good at evaluating these things, especially these coaches who have made it to the highest level. They are there for a reason because they are quite good at their jobs.
Gus Kaeding: But every once in a while, they'll raise a red flag and yes, it's okay to push back, but you better be pretty sure of what you're pitching. And that's a chance to go back and look at your own data. Maybe the underlying assumptions are wrong. Maybe it's something you haven't even thought of. So I will say throughout this whole process, I've certainly learned that these coaches have made it to the highest level a reason, and you can push against their instincts, but you should also listen to them as well.
Anthony: Yeah. Again, I think something that everyone in business should take back again, a senior executive with a lot of experience, a lot of gut it's an important check on whether a result we got from data was spurious or real. Let's talk a little bit about, I mean, I think something that a lot of listeners would empathize with is working with limited resources. I know that the US Ski and Snowboard Team is a nonprofit and as you pointed out at the very beginning, you don't have copious amounts of data. You don't have a team of 350 people collecting and analyzing data, but you do have a tremendous variety of different sports and teams and athletes, so there's a lot of resource there. How do you work with and have such amazing results in the face of limited resources?
Gus Kaeding: Well, one advantage that we do have you're right, there are limited resources, not only in terms of money, but also personnel. One of the advantages we have is that working in Olympic sport, you are a little bit, there is an end goal that is different than just the bottom line. So everyone is in this to accomplish something that goes beyond money. And that creates more of a community aspect. So when you are pitching these ideas to athletes, coaches, even people outside the organization, there is a greater sense to help. And I get emails every day from people outside the organization, just saying, Hey, I've seen the things you're working on. Would you like some help? And more often than not? I say no, just because it takes as long to bring someone up to speed as it does to do it myself. But one thing I've had a lot of success with recently is an intern program.
Gus Kaeding: And the way that's been structured is I just over the course of the year, I create a list of problems and there are things that may not be at the top of my list, but are really interesting to work on. And when I asked for intern applications, all I do is I present a list of problems. And I say, I think these are pretty cool. If you think these are pretty cool, you just get to choose which ones you want to work on. And I think interns love that freedom. And as a result, we've been able to get some really fantastic interns out of Harvard and UNC and just top notch colleges all over the place. And these are resources who come in, who are often really talented and niche areas and are able, I am able to use as a resource.
Gus Kaeding: And one of the things we've had success with is keeping in touch with this small community who have gone on to professional careers and PhD programs now. And when I have a tough problem, I'll ask the community and they'll come back and offer solutions, or maybe introduce me to someone who can help. And that's been a really fun part of this job and has kept me professionally motivated and learning new things, especially from some of the young ones coming up who take classes in college that did not even exist when I went to school, which was not that long ago.
Anthony: I love that. This idea of tapping into an intern community, especially in this space of data and analysis is a great one. As you point out students today are learning things certainly that even that I didn't, you didn't, and the ability to apply that, especially with such a laudable outcome helping the US Ski Team is, yeah sounds like a dream opportunity. Anyone listening, who is in school these days, you should yeah drop us an email. And it sounds like there's a great internship opportunity. Maybe we could cast our eyes to the future for a moment. And I want to ask you to make a prediction about the US Ski and Snowboard Teams performance in the Winter Olympics, but I will bite my tongue and instead ask from a data perspective, where do you see the US Ski and Snowboard Team going? What are some of the innovative things you're working on? How do you see the future of data and skiing and data in sports?
Gus Kaeding: Yeah. So actually it's planning and review time of the season. And the hot question this time of year, always by the board members, by the trustees is how many metals are we going to win next year? And I just did that analysis and I pitched it and I'll keep that under wraps for the moment. But I will say that the US Olympic Committee comes out with a total every year based on an ELO system, which is a chess rating system on athlete versus athlete, head to head match ups, but millions of them. And that is shockingly accurate.
Gus Kaeding: So when the US Olympic Committee comes out with their total, I would encourage everyone to look at that. But as far as future technology, one of the areas we're really interested in right now is, well, going back to the problem, getting from start to finish as fast as possible, we are restricted by what we can do in that time with the start and finish. And so look at Alpine Skiing where athletes often will hit 80 miles an hour down a two mile course in 10 degree weather with a 20 degree mile an hour headwind, very difficult to get data on a thing like that.
Gus Kaeding: So we have really, I would say two projects going to combat that. One is a really granular sensor that will go on to the athletes boot. And will deliver a really granular information about elevation, loss gain and, and to... Let's see... To the hundredth of a second, at least escaping the exactly. But then there's also pitch, roll of the ankle and it's really going to allow us to go understand what goes into a good and a bad Alpine turn, which then results in a faster or slower run. So that's approaching that problem from the sensor side and the challenge there is, it's something you wouldn't think is the challenge. The math is difficult, but very doable. It's the battery life. When it's that cold, you can't get a battery that lasts for more than 10 minutes.
Gus Kaeding: So that is something we've been working on and I think are progressing on, but the other end of the spectrum is using video analysis. And again, you're not in a set environment. So very difficult to have a video of an athlete going at 80 miles an hour for two miles. So what we have to do is use closed environments where we can, and as things like computer vision really continue to progress, we are cautiously waiting. I would say for the prices to come down a little bit, to roll out in any sort of mass use, but are now better testing with specific athletes and more closed environments and using video technology to understand what goes into pose estimation on a good turn or a bad turn, and really looking at how that impacts the start to end time.
Anthony: Fascinating. So really it sounds a big bet on IOT and really ramping the collection of data, new data sources, and for figuring out how to bring all those data sources together. And again, I love the way it always connects back to the key overall objective, which is how do we best represent this country in the Olympics and maximize the number of medals we bring home. So Gus, I want to thank you. It's been a great conversation. There's a lot in here for listeners to take away as they approach and attack some of the key data challenges in their organization. I want to thank you for your time and for some amazing insights.
Gus Kaeding: Yeah. Thank you, Anthony. Thanks for the time. And I'll be a listener from here on out. I've really enjoyed the previous episodes. So congrats on the success and yeah thanks.
Anthony: Good luck in Beijing.