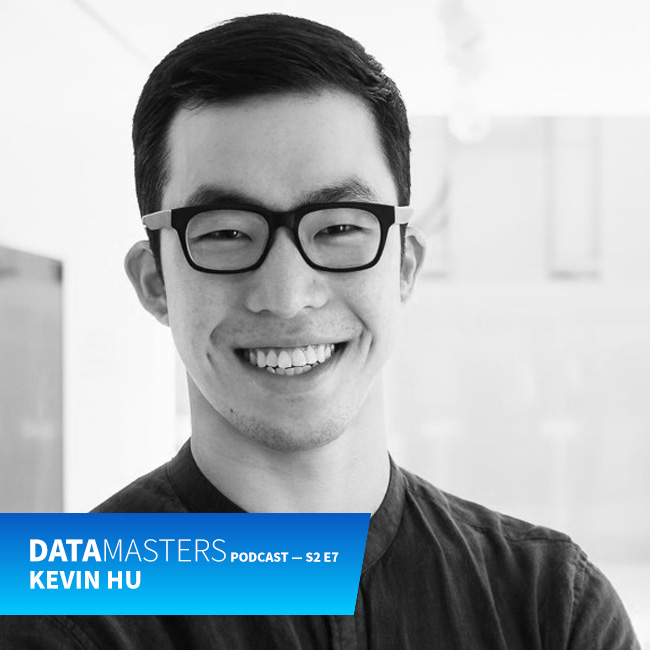
Turning Up The Volume On Silent Data Bugs
Kevin Hu
Anthony sits down with Kevin Hu, CEO and co-founder of Metaplane, a new data observability company. The two discuss whether bugs exist in data, what Kevin learned from Y Combinator, and what the kids are studying these days.
I'd rather read the transcript of this conversation please!
Anthony Deighton
Welcome to the DataMasters podcast. I'm your host, Anthony Deighton. Today's episode is a bit unique because it's the first time you're recording an episode in person, so we'll see how this goes. I'm excited to welcome today's guest Kevin Hu, Kevin is the co-founder and CEO of Metaplane Data Observability Platform that helps data teams be the first to know of data quality issues. Companies like Imperfect Foods, Drift and Reforge use Metaplane to increase trust and save engineering time. Kevin launched a plane out of Y Combinator, which we'll talk about in this episode before Metaplane, Kevin researched the intersection of machine learning and data science at MIT, where he earned his Ph.D.. Welcome to the office and welcome to the podcast, Kevin.
01:33 - 01:41
Kevin Hu
Thanks for having me here. No, just chatting casually over burgers and fries or something that you definitely can't do over Zoom.
01:41 - 01:47
Anthony Deighton
So I appreciate that is calling the Zoom version of burgers leave much to the imagination.
01:49 - 02:03
Anthony Deighton
So maybe we can start a little bit with your background. So an MIT Ph.D. maybe share a little bit about how you ended up at MIT. What you were studying and researching there?
02:04 - 02:52
Kevin Hu
I started at MIT as an undergrad. I studied physics at the time, of course, as they would say. And I was such a nerd about thermodynamics and quantum physics was what I was focused on. And what got me interested in data actually starts from the Gauntlet course at MIT Physics, where every student has to take an experimental lab course called J Lab, and this lab course was notorious.. They would say, you know, you have 40 hours of coursework in the week I allocate 30 of to J Lab, so as brutal as known as the feeder course. And surprisingly, I thought it was very easy, but I don't think I'm a particularly good, you know, physics student.
02:53 - 03:39
Kevin Hu
What it came down to was two things. One is that I had a great partner in international physics, Olympic gold medalists, which I'm sure you know, carried a lot of the team there. But the second part, which is very interesting, was the way the course was structured is every two weeks you have to replicate a Nobel prize-winning experiment. And then the second week you have to write the paper and then make the presentation. And when I stepped back and looked at the class, I realized while everyone does the experiment in the same amount of time, what takes the most time is collecting and analyzing and interpreting the data and writing it down in a way that can be communicated to the teachers. That's what people were working on late into the night.
03:40 - 04:20
Kevin Hu
And also when I realized, Wow. The way that we work with data is such a bottleneck, right? In parallel, my sister, who is a biologist who was going to her city at Stanford at the time, was messaging me, Hey, you know, I have all this experimental data from five years of grad school. Can you help me analyze it? I was like after five years of hard science. The problem is that you can analyze your data and are this is a little bit ridiculous, which is how I got into the Ph.D., where I had a great advisor, Cesar Hidalgo, and did research for six years on how we can augment and automate the data science process.
04:21 - 04:53
Anthony Deighton
Interesting, so like and I guess a very common experience is that you have a sort of personal experience with in this case, getting meaning, finding meaning in data and then a particularly difficult problem in academia because most cases in academia, you don't know if there's any meaning in the data, like at least in business, we can say, you know what? Our sales are, right? We can. We sort of know that they are something. In many cases, you may run an experiment and there may actually not be anything there.
04:53 - 05:27
Kevin Hu
Right? That is a great point. And I think. The challenge of data science is not necessarily making the data have meaning when it doesn't have meaning. But you get to the answer as quickly as possible with as much confidence as possible, really trying to increase the iteration speed between, you know, something with a domain knowledge, having a question and then proceed with the answer, whether that's, you know, five minutes for Zone, who has no well-structured, structured data and is familiar with how to you work with it or five days or five months, I think makes a huge difference.
05:27 - 06:10
Anthony Deighton
All right. So we can increase the cycle time, the pace with which you're looking at managing and understanding the data that actually has in academia has significant benefits. And obviously that that translates pretty directly into that into the business world. 100 percent. So the other thing I used to have this theory that said, if you want to understand what's happening in the world, go look at what undergraduates are doing. So, you know, my first experience with a web browser happened because we were introduced to it as undergrads. We we even did things in undergraduate with some sending emails and stuff like that, which were at the time, relatively cutting edge.
06:11 - 06:34
Anthony Deighton
So I'm curious from your perspective, as someone who's a bit closer to what people are studying in academia. Are there technologies and research topics within academia that that people should be aware of at art? Because of course, they're not close enough to academia within our particular field of working with data?
06:34 - 07:30
Kevin Hu
I was lucky to be on. The very early side of what I think is a super exciting trend, which is trying to apply machine learning to data science processes. One of my thesis readers, Tim Cross-code, for example, has amazing work on trying to train deep neural networks to create indices for databases for indices or just no statistical structures that roughly mapped some input to your location. And you were saying, you know, given a bunch of queries, can you learn that function? And I would say on many components of quote unquote data science, from collecting data to managing it and reconciling everything to visualizing there are some approaches where you can apply machine learning or against like a large dataset to augment that process.
07:30 - 07:38
Kevin Hu
I'm particularly excited about the work that's going on in automated visualization. I find a little bit biased because that's what I was doing research on.
07:39 - 08:33
Anthony Deighton
But I think that's a really interesting point, which is that the if you before machinery there was always a very structured algorithm to, or one might say, a rules based model for performing any sort of work with data or, for that matter, work in the real world. So I always think about this analogy to the self-driving car, which is the mechanism of making an automobile workers to literally provide direct input to the direction and speed of the car through the accelerator and the steering wheel. And the great insight of Tesla, for example, is that no, actually, we can just get a sort of general idea of where you'd like to go from the from the driver of the car drive itself. So it feels like maybe this trend of machine learning is actually being applied much more generally across all kinds of domains.
08:33 - 08:57
Kevin Hu
Definitely. And I think some of the driving cars are a great example of like a human pain. I don't want to have to do that commute every single day to use like a well-defined problem. And it's a very, very challenging problem for sure on the case for driving. You can have that many mistakes. Oftentimes in the data science world, you can have a couple of false positives. It's not the end of the world, but the knowledge is definitely there.
08:58 - 09:18
Anthony Deighton
Yeah, yeah. Luckily, the the domain of data technologies is both probably easier. By nature, it's it's a data driven problem was, you know, driving is a very real world problem. But but also with the risk of failure is maybe a little easier, that's for sure.
09:19 - 10:11
Kevin Hu
And I think of some work, really exciting work happening on semantic type detection. Oftentimes, not all of these data technologies are based on the assumption that you know what the data represents and currently in a database, if you put in a column of latitudes, for example, it will be our float or a decimal, which kind of makes sense because that's how the data is being represented. However, the moment you know that it's a latitude and longitude, the possibilities are much wider for how you can work with this data. Now, I know that is a location. And so that's another field I'm just very excited to see where again, like the cost of being wrong is not so high and the benefits of being right is, as you point out, tremendous.
10:11 - 10:27
Anthony Deighton
So now they know what you're dealing with from a data perspective and sort of latitude wanted to make a lot of sense, but even things like, you know, sales, right, which which will have a very specific range and domain that they're going to and they should be represented, possibly in a cryptocurrency and things like that.
10:28 - 10:57
Anthony Deighton
So I'm shifting gears slightly. So you're at MIT, you do a PhD. I happen to know that what you're supposed to do when you do it is become an academic and become a teacher. You are not. You started a company Metaplane. So maybe before we could dig into what Metaplane is and how you started it? Maybe start with a more basic question, which is why start a company and why not go be an academic?
10:58 - 11:34
Kevin Hu
Well, I kind of accidentally stumbled into the team. I never intended to do it. I just knew that in my 20s, I wanted to have the best boss that I could. And my undergrad advisor at the time, Cesar Hidalgo, was such an amazing mentor to me. For example, he coached me through finding the first research projects. He made connections with people like Steven Pinker that I had no right talking with. And he eventually gave me a book and I read this book.
11:34 - 11:56
Kevin Hu
The next step for you to progress is to learn how to tell a story like Steven Pinker can. It was the blank slate. And this is you, my undergrad advisor. I wrote, Who am I to deserve this time? And I was very grateful for that. I just realized I want to keep learning from him, which is why I stayed on for the Masters and eventually for the Ph.D..
11:58 - 12:25
Kevin Hu
What led me to industry was the recognition that I wanted to. Impact as many people as possible, and right now when it comes technology rather to value chains that are so horizontally applicable when a software or software is in the world and the other one is data, which kinds of one company out there isn't trying to collect data, know, improve their business processes, improve decision making and.
12:26 - 13:06
Kevin Hu
I feel like sometimes where you have the most impact oscillates in academia. Some of the most brilliant database research. The most impactful database work has come out of academia, but recently, right, it's been an industry, right? New data warehouses have been developed that are going to touch a billion people. I think now we're in a time where the pendulum is more on the industry side, where you see people not choosing to not go into academic paths and academics themselves started. Companies are leaving academia entirely. Because the technology is kind of ready and the industry is ready to build these tools.
13:06 - 13:28
Anthony Deighton
Yeah. So it's an interesting point that historically we've seen that what we might call fundamental research occurs in academic settings, right? And your point, I think, is that no, I mean, obviously that still still occurs. But potentially we should think about industry as a source for fundamental research.
13:30 - 13:44
Kevin Hu
I think that's totally right. Well, industry is definitely becoming a source, especially on like machine learning research. Are you see a large software companies building up enormous, well-funded, prestigious research teams?
13:45 - 13:46
Anthony Deighton
Yeah, interesting.
13:47 - 14:05
Anthony Deighton
So when you go to start medically and you also do something maybe not unexpected, but something very specific, which is Y Combinator, so maybe you share a little bit about what that is or how that path works, because not everyone may know that and then maybe share a little bit about why you chose about.
14:06 - 15:01
Kevin Hu
Y Combinator is a startup incubator where they give you some money for equity in your company. You go there for three months and they try to accelerate your company as much as they can. Some Y Combinator companies include Stripe, Dropbox, DoorDash, Coinbase, Airbnb. The list goes on. They've had a huge amount of IPOs just in the past year. We've tried to get into Y Combinator many times, we actually applied four times, and it was on the fourth time that we finally got in. And that means our three times we had to fly from Boston to San Francisco just for a 10 minute interview to be grilled. And then we just fly back and we get an email saying, this is why I didn't get done. But eventually we got into the W20 batch and it probably was the highest leverage three months in our startup's life.
15:04 - 15:37
Kevin Hu
I see really. I think back to that time, and I really distill what they did into three buckets. One is they had guest speakers every week. Our first guest speaker was the founders of Airbnb. And then the next week after that was the founder of segment. They recently sold to William, and they kind of gave you different success stories and made you realize that there isn't one formula for startup success, right?
15:37 - 16:08
Kevin Hu
Airbnb the founders had so much conviction and not conviction for them to sell cereal to survive. The stripe founders were more like they pivoted multiple times until they found something with product market fit. And you see no start as far on both sides of that spectrum, right? Airbnb, Coinbase high conviction segment amplitude retool. Kind of went all over the place until they found it there. So that was one big takeaway is that there's no one right way to build a company.
16:08 - 16:54
Kevin Hu
But the second takeaway, which I'm sure is not news to you or your listeners, is that there are common failure patterns, but there are ways to not build a company. And I would say that the famous y'see, advice talking to your users versus, you know, listening to investors or competitors too much shipping quickly reducing your burn rate are all pieces of advice that counter common failure patterns, increasing your burn too much, talking to the wrong people by shipping too slowly when as a start up, your only competitive advantage is time. Basically, you can outstrip your competitors. I mean, those two things were huge boons to us, and we still think about them all the time.
16:55 - 17:08
Anthony Deighton
So is it fair to say that Y Combinator sort of provided obviously a platform to launch the startup, but also sort of founding principles that helped guide the experience?
17:08 - 17:29
Kevin Hu
That's fair to say and a great community. We keep in touch with a lot of people from our batch, many of which are also startups in the data space like air bright and high touch are two startups in the modern data ecosystem. So I think the principles in the community are well worth the equity.
17:30 - 17:57
Anthony Deighton
And then if there was a listener listening who was considering doing a startup given where your process through Y Combinator to launching another plane? Are there advice you would give them? Not another one is going to be able to get into Y Combinator is run one challenge, but anything you took away through the process that you believe is advice for them.
18:01 - 18:36
Kevin Hu
I would say that. Why is a goal in itself? I know many people tried to get into, y'see to have that on their resume, but you get in when you build a good company and the end state isn't like other devices like you continue building a good company. When the fundamentals are solid, everything else will fall into place and we have to keep reminding ourselves of that too. I think one challenge of building a startup is you got pulled in a billion different directions, and yet you can only make one or maybe two moves. It's making a decision that's tough.
18:37 - 18:54
Anthony Deighton
Yeah. And the other point about I see is also really interesting because the same is true in a later stage. So we used to, you know, people think of an IPO as a goal. And one thing to remember, of course, is that no, it's just a step on a journey.
18:55 - 19:24
Kevin Hu
If you read through some of the, y'see, recent blog posts, they'll tell you, like DoorDash from where I see the IPO. And it's like, you're saying it's one continuous journey from two people in a cramped Mountain View apartment to you, a thousand person IPO coming in and beyond. As we know, companies grow significantly past, I feel like you said, and hopefully so I thought that would be certainly the goal.
19:25 - 19:38
Anthony Deighton
And especially if you're trying to build a great company as opposed to just trying to do a startup like just say that the goal is not a startup, the goal is a great company. Right, exactly.
19:39 - 19:57
Anthony Deighton
So maybe speaking of startups and great companies, let's talk a little bit about medaling and maybe start with just a quick overview of we talked about data observability and that the broad challenge maybe give listeners a little bit of an understanding of what the company does.
19:58 - 20:18
Kevin Hu
But a plane is a data observability tool that plugs into your data stack, for example, warehouse like snowflake transformation, total activity dashboards like liquor and simply, we let your data team be the first to know when something goes wrong and what the impact is.
20:19 - 20:58
Kevin Hu
So why is this problem important? Let's go back a little bit. Where in data teams today? Frequently, did teams are the last to find out about data issues? Right there go to their slack and the head of marketing says, Why does this liquor dashboard look weird? Why is this table not updating? And we feel like this is kind of like a systemic problem where the amount of assets that you can create as a data team, the tables dashboards quickly grows. Over time, you get hundreds or thousands of liquor dashboards, and there's no way that you can audit all of that.
20:59 - 21:23
Kevin Hu
So what we're seeing is companies are taking advantage of technologies like snowflake and DLT tools to store and model more and more data. But there's a little bit of a ticking time bomb because there's it's only a matter of time until one of your stakeholders starts to lose trust in that data. The purpose of a plan is to make sure that that doesn't happen.
21:24 - 21:27
Anthony Deighton
So at its most basic, you're selling trust.
21:29 - 21:40
Kevin Hu
We're trying to sell trust. Exactly. Because once you have the data in place and it is being used, the most important pillar is that the data is trusted.
21:40 - 22:29
Anthony Deighton
And then the the the opposite of trust is when there's a systemic failure and you are the one who discovers the failure is the one consuming, for example, the dashboard or consuming the data. And it feels like the analogy here, which I think you make very explicit, is the analogy to how software development is changing. So historically, when we built software, the way we discovered a problem is when we shipped buggy software to the customer and they came back and said, My God, this thing's junk or it doesn't work. And then we work backwards to figure out how to fix that. Is that a fair analogy?
22:29 - 22:59
Kevin Hu
I think it's a it is the analogy where not only an observer ability, but in other parts of the data stack, for example, the new term of the analytics engineer we're recognizing as a community that while there are. Appreciable differences from the software world that there is a lot we can learn from the software engineering best practices that have developed over the past few decades with observability in particular.
23:00 - 23:41
Kevin Hu
Like you said, you used to ship, you know, an end point, maybe a heartbeat. Check on it. Call it a day. Nowadays, imagine walking onto an engineering team and not installing Datadog or a signal affects the. That's a little bit unprofessional. You will be laughed out of the room. And yet operating in the dark is unfortunately how many data teams are operating today. The no fault of their own right. You have so much on your plate, and the technology just isn't quite there or easy to adopt for you to have that sort of visibility, but hopefully things will be changing in the coming years.
23:42 - 24:31
Anthony Deighton
So one of the reasons that we've seen such a revolution in the software development space is that we have significantly different architecture in place of very specifically a card architecture that we have data sources that live and breathe in cloud environments. We have access to essentially infinite compute storage at very reasonable costs. And certainly, that wasn't true five, 10, 15, 20 years ago. You know, I know for better playing snowflake is a big partner. How do you see for? How do you see the infrastructure of data ops and data engineering changing that then enables a solution like better?
24:34 - 25:16
Kevin Hu
Going back to what we were saying earlier about why now is a great time to start a data company to have impact on a billion people or more. Part of it is the centralization where some of our smallest customers, one person data teams are using snowflake DB10 liquor. Some of our largest customers and thousands of employees have the exact same data stack. Snowflake debutant Flickr This would have been possible even just a few years ago to have a small set of integrations with so much gravity in the data stack that you can build integrations with and address a large market.
25:18 - 25:48
Kevin Hu
And the ability to infinitely scale compute for observability in particular has eliminated the tradeoff between data quality and data performance in previous generations of database systems. You couldn't have hourly checks on your data because you have hourly people using your data. Way want to have this huge table scan bottlenecking my API dashboard? Now that's no longer an issue, right?
25:48 - 26:16
Anthony Deighton
So you can your. Infinite performance on infinite compute at the ready to be able to do the work you need to do to observe the platform and not get in the way of actually using the platform. Maybe you could share at a very practical level some customer example or how better it is being used, in particular, how a business user would experience the benefit of a system like this.
26:18 - 27:05
Kevin Hu
Well, one thank you, papa, more for Moore's law that makes it possible for us, we have customers across all sorts of verticals, from health care to being a business to fintech companies. And one common pattern that we see is lack of late data, where data in snowflake is not being refreshed and a business user should know that the dashboard that they're looking at is 12 hours late. This happens almost across the board with all of our customers. And with with our plane now the business user has done the first and now because they ask, why isn't this being updated with Metaplane?
27:05 - 27:41
Kevin Hu
We have anomaly detection systems based on the freshens of raw data accounting for a seasonality and trends. And we alert you when you no longer than expected. Time has elapsed since your data has been updated. So practically we send a stock alert or a PagerDuty alert to our customers, saying this table has not been updated. This is what we expected. These are the downstream tables and dashboards that are impacted. And if the data is available, here is who you should know about that.
27:41 - 28:23
Anthony Deighton
Got it. So the the general concept here is the data pipeline is something's broken in the data pipeline and the results of that as a downstream impact on the business. Their dashboards aren't up to date. They complain about that. And again, bringing these two ideas together software development and this data pipeline. This feels like what we might in software development call a bug as a bug. And and now the detection of the bug occurs when the software doesn't compile or we can't ship. So do you have the same concept in data observability of a bug? Is that a fair analogy?
28:24 - 29:02
Kevin Hu
You're exactly right. Data can have parts and that operates orthogonal to software parts. Of course, they're related. Data is often generated and manipulated by software. But all of your systems can be green. Write your snowflake has operations running. It's running. You're just missing half of the data and it is 72 hours late. The data can definitely have bugs, and oftentimes it's silent. It kind of like sneaks its way past your systems until it gets in front of the eyeballs of someone who knows what it should look like. Again, the stakeholders.
29:02 - 29:42
Anthony Deighton
So this idea of a silent buck is a really interesting one, because maybe to put words in your mouth that one version of the problem of data observability is there is no data, but that's clearly a. But another it may be more you're more, more dangerous. One is the silent problem, which is there is data is just incorrect and within that as well, there's less data than we expected. But it could also be that the data is just weird. Like you're getting all negative sales, like, oh, you're getting all sales of only one product or something like that.
29:43 - 30:07
Kevin Hu
We don't want it to be silent. That's the thing. Right? All negative sales. We want to be very, very loud. And that's part of what the observer believed tools are trying to address. But I think it's also part of a larger organizational issue where everyone is has some skin in the game when it comes to data quality.
30:08 - 30:09
Anthony Deighton
Yeah, interesting.
30:10 - 30:25
Anthony Deighton
So the the idea of so it's like you're almost like giving a voice to the to the data so that you don't have to rely on the end user in this case, the business professional to raise the alarm.
30:26 - 30:50
Kevin Hu
One analogy that a friend, Gordon, one former VP pick HubSpot, says it's like data is kind of like food service where. You need to source the ingredients. Come up with the recipes, you have your kitchen cooking, you have the waiters serve the customer and then the customer is there for at least a review that might come back.
30:51 - 31:38
Kevin Hu
There's so many steps along the way where something could go wrong, and if there is some sort of a data quality issue, for example, food poisoning, you might not find out immediately. You just know for sure that that customer will not be coming back to your restaurant without some major changes. That is just different from the software world, a little bit where the idea of lineage is so specific to the data world. Of course, there are infrastructure dependencies within, you know, an application. But one particular piece of data tracing it all the way through to the end user is kind of a thing that's unique to the data world and a critical step to finding and squashing data bugs.
31:39 - 31:40
Anthony Deighton
Interesting.
31:40 - 31:53
Anthony Deighton
And then sort of linking this back to where we started with your academic research on machine learning, have you built machine learning into better plane or is there a machine learning component or how does that play?
31:54 - 32:40
Kevin Hu
There are. Time series analysis components, and there definitely are machine learning aspects. We haven't we've only started scratching the surface to understanding like how every customer can help other customers. Of course not by sharing their data or even sharing their data. But the amazing thing about our world, the data world, is that the same models that help imperfect foods. E-commerce company sends you boxes of ugly vegetables and fruits can also apply to you like a credit card company. There is very similar patterns that you see in their data, and that's where machine learning can come into play.
32:41 - 32:54
Anthony Deighton
Interesting. So the anomaly it's really about anomaly detection and what defines an anomaly is almost independent of what the actual underlying data is. It's.
32:55 - 32:57
Kevin Hu
Separate, but similar. For sure.
32:58 - 33:29
Kevin Hu
Where on the most atomic level anomalies are anomalies. Just the time series. But if we want to become more sophisticated as an observer ability to, we have to start recognizing the semantics of the data. Go on to your example before that, once you know that it's a latitude or a monster, this refers to you cells. You can start applying other sorts of models and rules on top of it that are more specific to that user.
33:30 - 34:02
Anthony Deighton
Interesting. So as we sort of wrap up a little bit. So, you know, you've gone on this journey from academia Ph.D to to a startup, but anything you want to leave listeners with as it relates to your career journey and the future of where do you see data ops and software or data observability going in the future?
34:03 - 34:58
Kevin Hu
I would say. One that as we build tools, we should challenge ourselves to think, what does the data world look like in 20, 40 to 20 years from now? What tools are there? What jobs are people working? And once you extend out the data to that time horizon, things start getting a little bit interesting. Right? So probably be here databases, probably the same databases we have now. Not sure. But you have to kind of boil it down to the fundamentals of you have sources generating data use cases that can use that data. Everything in between is a bit of a wild west. And what we have to do with the modern data stack could be the optimal configuration for where we are along the curve of Moore's law.
34:58 - 35:22
Kevin Hu
But 20 years from now, that isn't necessarily the case. But why we're so excited about observability is we're comfortable saying that at 20 years. Any company with data will have full visibility into where it came from, what the impact is and how it has trended over time. So this idea of losing data trust is no longer even an issue.
35:23 - 35:51
Anthony Deighton
Yeah, and maybe to extend that thinking a little bit. One thing I want to say is that at its core, every business is a data business. And so if you believe that it is at a fundamental level that you know you're not really a retailer, you're not really a hospital, you're not really a reasonable company at its core, what you are is a data pipeline without observability. You're really not managing that core asset of who you are as a business, which is your data.
35:53 - 36:14
Kevin Hu
That is so you're right where and we had to treat it with as much respect as we have begun treating software, right? We, you know, software is a revered field in our world now. But that hasn't always been the case. It used to be part of body function like our costs on our side.
36:14 - 36:48
Kevin Hu
Business aside, exactly. And I think we're just starting to see data emerge from the primordial ooze in a lot of companies to become a major competitive advantage. And one last time I would leave to you, your listeners, I imagine our many data practitioners is. If you have the itch to start a company, I would also encourage you to do it. You know, of course, for me, I've been super fortunate to have the resources along the way to support me on that journey.
36:49 - 37:14
Kevin Hu
But at the end of the day, what improves the state of technology today are practitioners building what they wish they had. At their current roles, and I have full confidence that they take the leave right now, the market is very friendly towards founders and it's like such a good time to learn and velocity is incredible. So I'd encourage you to take the leap.
37:14 - 37:51
Anthony Deighton
And if you need any help, just as I'm an email to excellent now, I think that's such a brilliant way to have it. Because if you are the archetype of that, someone who starts their career in academia, working with data frustrated by the ability to work with and manage that data, get inside out of it and you solve that problem by starting a company to fix the problem, which is perfect that I agree with you. Every one of our listeners should do the same thing. So with that? Kevin, thanks for making the time and joining me in person for our first live and in person for the last week.
37:51 - 37:52
Anthony Deighton
But such a pleasure.