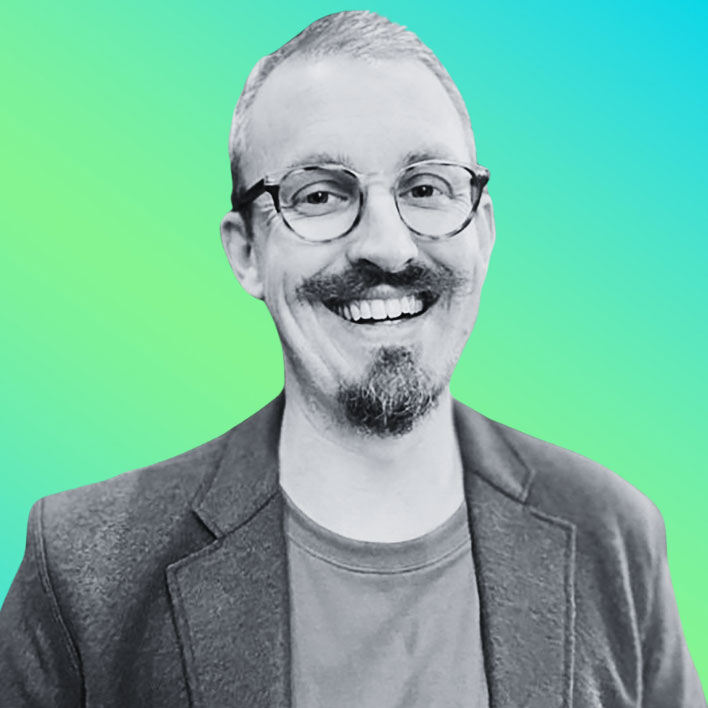
Integrating AI and Data Into Executive Strategy with Austin Tidmore
Austin Tidmore
In this episode, Austin Tidmore, VP of Data Innovation at ASGN Incorporated, discusses how leveraging data and AI can drive intentional business innovation. He highlights the importance of augmenting human capabilities with AI and explores the intersection of data and enterprise strategies to enhance business efficiency and value.
I'd rather read the transcript of this conversation please!
Austin: [00:00:00] do separate the idea. Of innovation from disruption. I think disruption, as a broad stroke idea doesn't. necessarily fit into the culture or the ethos of a company. And while I will admit as a consultant, I might've gotten a little loose with using the word disruption, as I was talking with perspective clients about really interesting technology and use cases, back to Data Masters. My name is Anthony Dayton, and we have the pleasure of having Austin Tidmore joining us today. Austin is the Vice President of Data [00:01:00] Innovation at ASGN Incorporated. I'll have him introduce himself, but ASGN is a, Company that really focuses on I. T. business solutions, both in the government and in enterprises.
Anthony: And Alston leads data and A. I. strategy for the organization across their five divisions. Welcome to Data Masters, Alston.
Austin: Thank you so much, Anthony. It's a pleasure to be here. I feel among, the greats being on this podcast.
Anthony: Excellent. So maybe if you don't mind just start, I know you've had a wide and varied career currently, obviously you're at ASGN, but maybe start us off with. Sharing a bit about yourself and how you've come to this place at ASGN. a bit about your role and the focus there.
Austin: Yes, it sounds good. I'm here in the Dallas Fort Worth area, a Texas native working for ASGN as the VP of Data Innovation. Certainly a more unique title at a rather large company that not a whole lot of people are familiar with, but they are familiar [00:02:00] with our brands. is, is a conglomerate of like you mentioned, five. Divisions or five companies that each operate really well in specific sectors of the talent and professional services industry. So we do everything from temporary contractual staffing to professional services and consulting work on both the federal and the commercial side. and like you mentioned Having my focus as data and A.
I. across the five divisions is a relatively new tactic or even strategy at the company based on our strategic objectives and where we're headed the idea of doing things more collaboratively and in a more unified sense luckily has our executive team thinking about that. Leading that strategy with data and artificial intelligence.
And so they brought me in to work for our Chief Innovation officer to really start building that roadmap of what does it mean to [00:03:00] unify our business with data and artificial intelligence capabilities that help us operate more efficiently, but also add tremendous value to the clients that we serve.
Anthony: I love this idea of connecting innovation to data. I often talk about this idea that. Every business strategy under the covers is really a data strategy. There's either you're missing the data you need to implement that strategy, or you need to analyze it in a new way. The data is really the underpinning of most business strategies.
But I think that not to put words in your mouth, but your idea is that maybe innovation be powered by data, really thinking about data in new ways.
Austin: I totally agree. I was really fortunate to get a very relevant degree back in the day from the University of North Texas. I joke that I'm one of the few, probably, people in the business world that get to leverage 80 or 90 percent of their undergraduate degree, because it was very relevant focusing on what they called [00:04:00] at the time.
well, Less about machine learning and more focusing on decisions under uncertainty and decision trees and a lot of quantitative methods to formulate business strategy. So I got this great foundation and then throughout my career touching various kinds of technology, really witnessing at the forefront. the evolution of what was considered cutting edge and innovative at the time, I think, really helped me keep my finger on the pulse of, organizations that I'm working for, organizations that I was consulting through the business intelligence boom, and through the cloud computing rushing onto the scene, and Hadoop, and big data, and then machine learning really keeping my finger on this pulse of, What is relevant for an organization?
And that answer is different depending on where the company finds themselves in their own life cycle and their industry. And it helped me take a lot of those new technologies or new innovative [00:05:00] solutions and apply them in a way that really tries to maximize. the value for my business. And in some instances dashboards mobile delivered dashboards was very innovative.
It was a very transformative thing for an executive team to have their operational data at their fingertips. Even if maybe in some Silicon Valley garage somewhere, something far more innovative from an objective standpoint was being done the fact that, the company that I was working for realized so much value from that solution, I think helped them innovate as far as their business goes.
Anthony: maybe, sorry to go backwards a little bit in this, but you mentioned that you have both been a consultant and then now more recently taken over in a CDO role. Maybe talk a little bit about that career transition because I think many listeners have spent time maybe working in consulting today and thinking about, Taking on like a CDO role or an executive role inside a company or [00:06:00] thinking about, what that transition might look like.
You've done that, maybe share a little bit about how that looks and how, what are the differences and benefits and cons to it.
Austin: Yeah, you know, I, um, will say just for clarity's sake that my goal with The VP of data innovation role that I'm in is to prove beyond the shadow of a doubt how valuable data and artificial intelligence are to an organization that they deserve so much strategic focus that it should be an executive level role that, you know, gets direct attention from the board.
But I'm not there yet, but certainly I hope to be someday, I Joke that every year that you spend in consulting or professional services is worth two years of experience on your resume. And that really came to fruition in a couple of different ways. First of all getting exposure to typically clients across multiple industries. If not concurrently, certainly, back to back, as well as getting [00:07:00] exposure to a variety of technical environments, helps you develop this framework around assessing, And identifying and then, of course, implementing technology and technological solutions that I think it's really hard to cultivate outside of that.
And, of course, I won't say that you can't cultivate it, but I certainly witnessed firsthand this, I suppose this career trajectory that grew a lot faster than I really anticipated because of that problem solving framework around again, technologies and use cases and business strategies so I mentioned having a front row seat to a lot of innovative I guess eras of data and A.
I. technology. I started out in the data warehousing world really learning what it means to consolidate data into a single source of truth and to build ATL frameworks around that and curate data for that operational descriptive reporting. [00:08:00] And then as Major cloud commercial platforms came on to the scene and unlocked the ability to access. larger scale compute power relatively more, easily than it was in the on prem days. business leaders started talking to me and asking questions about if we can build analytics on this, maybe on this small section of operational data or on this one process, what would it look like to do it at a much larger scale or maybe in a much more real time fashion.
And so trying to answer and meet the needs of businesses and of clients in that regard, helps me deploy some of the first spark clusters, in in organizations and help data teams and engineering teams make this cognitive shift. From pretty, pretty data in rows and columns that is all neatly organized in maybe an Oracle or a SQL server database somewhere to thinking about massive distributed data sets of [00:09:00] JSON that is has to be very delicately, I will say brought together in this new big data context.
And it's really exciting. To see the proliferation of cloud compute really keep building and allow me to scratch that itch that I had back in my degree days of quantitative analysis and and then moved into applying a lot of those techniques to the data that I was already working with, maybe in a business intelligence context or in a Spark context and what would become machine learning models on data.
And that just so happens to really come to the forefront, maybe even just 10 or 15 years ago. In terms of being extremely relevant because it was more approachable, thanks to. Advances in Cloud Computing. And of always striving for leveraging technology and techniques to make data, to make this capability of analytics as maximally relevant to the [00:10:00] organizations that I work for.
Anthony: you said something there that I think is really important and you mentioned this idea that a catalyst for thinking about data in new ways was, your example, the cloud, right? And so all of a sudden what might have been a constrained resource, the amount of available compute or storage that constrained resource [00:11:00] was removed and all of a sudden, maybe putting words in your mouth here a little bit, but this constrained resource was removed and now all of a sudden freed you up to innovate and do new things.
And. I think it's very telling that innovation is, in a very literal sense, in your title, it's part of your primary job responsibilities. So I wanted to talk a little bit about what we mean by innovation in the context of data, maybe to muddy the waters a little bit and, you know, Put my own point of view on the table.
There's this concept popularized by Clay Christensen of disruptive innovation. And there's a lot to be said about it. But one of the things I think that I've noticed in disruptive innovations is that there's always some exogenous change, some outside influence That changes the dimensions of competition and allows for a new approach to be cost effective and and implemented.
And I'm bringing this back to your point [00:12:00] around the cloud. The cloud changed the economics of managing and handling and processing and analyzing data in a way that allowed you to do all kinds of new things. As you mentioned Spark, I mean, parallelizing things if you have to run the cluster yourself is.
One thing doing it, if you can leverage cloud resources is a completely different thing. but maybe you disagree that around what innovation means or maybe just to ask it as a question what do you think innovation means, especially given that's your primary job focus.
Austin: do separate the idea. Of innovation from disruption. I think disruption, as a broad stroke idea doesn't. necessarily fit into the culture or the ethos of a company. And while I will admit as a consultant, I might've gotten a little loose with using the word disruption, as I was talking with perspective clients about really interesting technology and use cases, but, I differentiate it just because like I said it's not necessarily compatible with every business model and with [00:13:00] every ethos of a company's culture or their board. And so I think to focus then on ways that we can innovate, which may lead to disruption in some regard, but really to think about innovation in terms of, essentially not fundamentally shifting our industry or our business, but looking at our value chain of what do we say we're good at?
What do we say that we? Add value and to whom along the operations in the day to day of our business then allows us to think of, okay, how can we maximize that value? Or how can we deliver the same in a more efficient way? is how I'm thinking of two sides of the coin of innovation. And in the consulting and the staffing industry, there is a lot of opportunity for innovation because of the very nature of our business is very manual. It's a very high touch as we think [00:14:00] about being the middlemen and women of a talent and labor supply pool with all of the demand of the countless companies in our economy who need value that a temporary worker can provide or the value that a highly specialized professional can provide that we then get to act as a conduit to bring that person from the labor supply into our client.
Anthony: Fundamentally, your business is. Human. I mean, It's really about people. And so I'm putting people matching people and talent requirements. And so to this idea of innovation and how to drive innovation, maybe, We could, or maybe you could rather, share a specific example.
Is there something that you've been working on that I think that illustrates this effectively and shows what it means to innovate take advantage of these technologies in the context of data, especially when fundamentally the process is really human?
Austin: You know, I'm looking at it [00:15:00] very carefully because I don't, prescribe to the idea that anything that artificial intelligence could do, or a data driven solution could do instead of a human should be pursued. I think I do very much ascribe to the idea of um, a human and their day to day job being augmented. by data and by artificial intelligence tools is the way to go. And luckily I'm here at a point in time where we're not innovating by switching from all paper resumes to digital, I'm a little further after that innovation, but and I think all companies in our industry find themselves in, at the crossroads of maybe a couple of. of different issues. You know, One is of course finding the best opportunity for a candidate based on their experience and based on their goals. And then obviously delivering human capital to a client that is the right skills and experience for goals [00:16:00] and the strategy and the tactics of that client and their organization. And there are a lot of quantitative ways that we can we can try to answer that question or again be that conduit between those two things. But it takes a lot of experience and it takes a lot of relational ability to be able to suss out those details. And pretty specifically we're Again, with the advent of with Compute and of, just great uh, advancements in the A.
I. sphere and with LLMs looking at those capabilities to mine a lot of that nuance from the profiles that we build of candidates and the profiles that we build of our customers. there's a knowledge base. That's really hard to build because, again, a lot of our interactions are very organic and they're very relational.
And so as I think about our innovation roadmap, there's a lot of value that we can [00:17:00] extract. from LLM approaches to finding the best fit for our candidates and for our clients in the short term. But there's also this journey that we're going on in terms of building a better knowledge base of data in a lot of different forms.
And I'd love to, if maybe, if you're interested, testing that out a little bit. More specifically, but building that knowledge base of data that helps us have the more relevant conversations with our customers and with our candidates.
Anthony: And to this question of LLMs I've seen, for example this idea that candidates are now using large language models to produce hundreds, if not thousands, of cover letters and, potentially even, tuning or customizing their resume to a specific job opportunity and, Maybe what is disruptive is the idea that you can do that at scale where historically in order, if I wanted to apply to 10 jobs and each application took me half an hour, that's a significant [00:18:00] allocation of my day to apply to 10 jobs today.
Maybe I can apply to a hundred jobs in 45 seconds, whatever it takes the LLM to churn through. Have you seen that impacting the business, or am I hallucinating and making stuff up? It is not actually happening.
Austin: that is a very thought provoking question and certainly have seen an influx of just volume and kind of in every sense of the word, I think directly attributable to a systematic ability to to generate and to tailor resumes. But that idea of. Brings us back to the human element of the business that we're in. And yes, LLMs and tools are able to help us mine the nuance a little bit of experience and of what we're seeing on paper. But it's going to take, a skilled recruiter maybe with the help of some A. I. based tools or some data tools to really get at how well the candidate tailored their [00:19:00] resume or their cover letter to the job description versus, you know, maybe fabricated a couple of bullets you know, in between to maximize. their relevance for the particular job posting. So, Yeah, it's something that we definitely are seeing experiencing from that data ingestion standpoint. And it will be really critical for us. To leverage technology in the best way to help us mine through, maybe I hate to call it noise, but essentially, you know, some of the more noise that's being introduced by such an influx of volume.
Anthony: Yeah, one hopes, at least currently, that a skilled recruiter can understand in a more nuanced way what somebody's skills are. And hopes and desires and dreams are and to the extent that the information they're presenting is entirely computer generated. Again, maybe I'll speak to my own personal experience.
My personal experience has been that the [00:20:00] kinds of text that LLM is presenting, Produce is useful, like in the sense of being technically useful, but also lacks a kind of humanity and lacks a and it comes across as a bit flat when we've tried to use it for writing marketing content, these sorts of things, I inevitably find we end up having to either completely rewrite it or certainly edit it heavily to be something that speaks in a voice It feels unique and not just tonally flat.
I'm not quite sure that I'm capturing it. I don't know. Has that been your experience?
Austin: I was some co pilot augmented tasks on my to do list this week that I was tackling. And I noticed, it's the power of suggestion often gets a little bit too literal in terms of. I'm always trying to refine my prompt approach and how I'm, I'm putting these prompts together to try to get the right information and I'm finding that they're very suggestible.
if I give it permission to ask me a clarifying question about something it may very well ask me a question. And then in my answer, it essentially gets [00:21:00] incorporated into the response verbatim. And I think I don't necessarily trust myself to, to give that entire. answers. So feel free to, be a little creative.
Anthony: doubt. And I also think maybe get your view here that there's a significant difference between the experience of large language models in a personal context versus a corporate context. And what I mean by that is large language models have been trained on data from the internet, which is by and large internet data, public data.
And yet what we do in the enterprise is. Deal with data that by its nature is not on the Internet. It's proprietary to us. It's our customers. It's our suppliers. It's things about our products. If I have a product company, it could be parts if you're a manufacturing company. And almost none of that data has been, LLM is having consumed that data by its nature because it's private to the company.
[00:22:00] And as such. I think there's actually a big opportunity in the enterprise to think about how to augment these models with proprietary information from inside the enterprise. We've often talked about this as giving large language models nouns, N O U N S, nouns for the enterprise, meaning when a large language model says, elephant, there's a pretty good description of elephants on the internet when it says, the ABC one, two, three product that it's never heard of, but the company knows a lot about that that's very different.
And so giving it that context, so it knows what that thing is, this customer of ours, the supplier of ours, that context and meaning is very different in the enterprise. I don't know if you, like in your business. I'm confident that there's a lot of proprietary information on the inside that these LLMs just don't have access to.
Is that fair?
Austin: That's a very fair [00:23:00] assessment and you're getting at something that is crucial for I think the success of of a data innovation leader is one of the drums that I keep banging here in, in my lengthy first six weeks on the job is, any company, any competitor of ours can say that they do A.
I. based recruitment or, whatever we might want to put on the bumper sticker, right? Data driven human capital. But if we can't activate our business this new capability or this maybe this new solution set then we won't really realize the benefits from this. And you hit that complex question very quickly in the enterprise context with LLMs because, the chatbot, generally available web version of of an LLM, you know, might be useful and, and help me in some regard or help anybody at our company in some regard. But really extracting the knowledge, the collective knowledge from the [00:24:00] company. Is, like I said, it pops up very early in the idea of all the things that we could do with this new technology and needs to be addressed. And one of the, there's a couple of initiatives that our teams are looking at that I'm really excited about of course I think the, maybe the first way to help solve that is, with this idea of a RAG or a retrieval augmented generation of the LLM to the user. But one of the things that I think is really making a lot of headway and showing a lot of promise is essentially creating agents or little applications, you know, that are generated or that are fueled by. LLMs, to do more than just receive a some input from a user or a prompt from a user. And then to find artifacts that are similar to, what that user has requested. And that's the RAG approach. But with agents what I think is showing a lot of promise is the ability to, mine a lot of that [00:25:00] data and information from a lot of different places very proactively.
You know, Earlier I was saying that we have a lot of data in a lot of different places. Of course like any organization, we have our structured data and certainly more than just one or two databases. And we have our unstructured data in certainly more than a few dozen SharePoint sites and Teams channels and things like that.
And. It's just not feasible to try to think of and code a solution around where all of that knowledge can live. You know, We're often trying to answer the question of hey, where have you attacked this use case with this technology in this industry before? And that information can come from a whole host of different sources that isn't a really conducive to a RAG approach unless we truly, really narrow the scope down. And LLM agents, I think, the showing promise in being able to almost autonomously with our guardrails, be able to go mine the information from various places to store it [00:26:00] in And highly retrievable formats, and then essentially work together to formulate that knowledge base. Our teams are leveraging some really interesting technologies.
I'm not sure how much I could or should get into it, but let's just say the very promising intersection of LLMs with graph. Databases, and kind of network based models that can represent knowledge, especially semantic knowledge in a really efficient way that all of us can, use and not rely on rags and some of the limitations around context.
Anthony: Yeah, I think that's 100 percent and maybe to be very direct on it, I think one of the things we've thought about at Tamar. Is thinking about that graph approach if you can resolve the entities in the especially proprietary data those really are the nouns in the enterprise and those become the nodes in your graph and getting those things right has a big impact on the quality of the graph relationships.
Now, you're actually viewing all of your [00:27:00] data through the lens of this correct entity which links these underlying sources. And it's okay that the sources are messy and disconnected because the entity relationships give it structure. And then using a graph as a mechanism of navigating that structure is a very common technique that's very powerful.
Yeah,
Austin: as I was You're working companies building business intelligence and that operational analytics, and then that started morphing into, I think we've all seen the maturity curve of going from descriptive to predictive and beyond. I always thought about machine learning and of course, under the umbrella of artificial intelligence.
So artificial intelligence by extension, but as a very linear as a very linear thing like, okay, we've got to. We've got to make sure that we get our data in order and it's structured and it's of high integrity because then we're going to build a data set that will train a machine learning model off of and the quality of that training process is going to hinge almost completely on the quality of the data that, that [00:28:00] was put into it. And so LLMs and where we've come over just the last just a handful of years in that regard has changed that paradigm a little bit because all that heavy lifting to create The large language model allows us to do things like semantic similarity and some content generation, you know, like you said, with, caveats, but content generation and for a few different use cases, you know, pretty rapidly, like we don't have to say, okay, in order to take advantage of this A.
I., we have to get our data house in order. But then again, talking about bringing very complex things up to the forefront to then let loose and an army of agents over your enterprise data does reintroduce that dependency of, okay, if it's, if you're going to have an agent to go mine, all of the clients that we've ever served over the decades of being in business I hope over the years, we've our different business units have called this same client, the same name and a spoiler [00:29:00] alert. They don't always do that.
Anthony: probably not. Yeah, which is, that's where we step in and, you know, hopefully give you a view of the entities across that. I wanted to make sure we left a little time to, for you to cast your eye forward and you, in particular, given your focus on innovation Where do you see this stuff going over the next, I think, of a reasonable time frame?
Three to five years? And you made this point a little bit under your breath, but I'll pull it up that we're not all going to be replaced by autonomous agents, with large language models. There's still a role for people and people doing real work. But, how risky is that?
Maybe that's true today and not true in the future, or am I, too futuristic? And we're safe, at least for the next couple of five, three to five years.
Austin: I believe innovation is a very intentional thing. I think and again, will admit a fault here for maybe perpetuating a stereotype about things being easy or simple. But since you've got me here, I'll [00:30:00] go, I'll just freely admit that. Innovation has to be a very intentional thing.
It has to be, again, where you're looking at the value chain of your organization and I, specifically identifying where you want to invest in because you believe it has utmost, return on the investment that you put into it. And so I understand that innovation and being a very intentional process is often, it is hard work. I'm certainly grateful that I didn't spend the hard work in developing and training the large language model that maybe we get to leverage every day. But again to build relevant solutions around this new technology has. Build relevant solutions around the technology in a way that really transforms the business, I think I'm always going to be looking as that is my end goal, regardless of how we get there.
this technology is impressive, and it's transformative sometimes it's, Humorous and entertaining to see in all of its [00:31:00] prowess, how it still can't count the rs in the word strawberry, so I think, it's going to take a significant amount of time for the reliability and the quality of especially, you know, large language models and their output to get to a point to where I think we are trusting them a little bit more freely. With that trust, and I think building relevant solutions around there will lower the guard a little bit for how the human and the machine does interact, because I think While it still has some novelty and while we still see it hallucinate and go off the rails a little bit in certain instances, that's really an it's impeding our ability to freely adopt it and to freely think, Oh, man, I could throw anything at this. and get relevant information about my business, to help me with my day job along the way. So I think we're still, over the next couple of years, we're going to still see a lot of focus on just the increased reliability of these kinds of [00:32:00] solutions. you know, Again I, throwing around this idea of a Jarvis, from Ironman for our organization. We'll try to exploit every possible capability, you know, at the forefront of these LLMs to, create something that is really holistic in its knowledge if not about the world, at least about our business, and so I'll look forward to ways that we can leverage and interface those LLMs and I'm not sure if I have a prognostication of where exactly it's going to be in maybe three to five years to enable that.
I think uh, it certainly will have made some of the use cases that we're struggling with today, more, more simple or at least more reliable.
Anthony: Yeah, no, I think that's right. And what I particularly appreciate about that point is this idea that at least in the enterprise these things become much more valuable when anchored on the data within the enterprise that, again, as we pointed out earlier, these models were not trained on. and so, sort of enabling or empowering them with that knowledge makes them more relevant and [00:33:00] useful in the context of, enterprise use cases. Austin thank you very much for joining us on Data Masters. It was a great conversation, really appreciate it.
Austin: Yeah, thanks for the opportunity, Anthony. I really enjoy it.