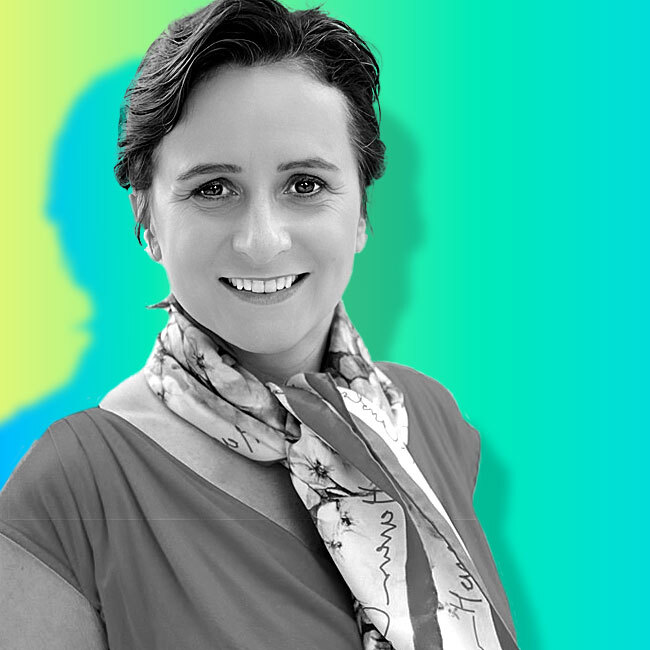
Ethical, Responsible, and Trustworthy AI with Dr. Eva-Marie Muller-Stuler, Partner in Data and Analytics at Ernst and Young
Dr. Eva-Marie Muller-Stuler
Before the advent of advanced language learning models (LLMs) like ChatGPT, Bard, and others, data science and AI were often seen as dry, serious fields focused mainly on questions about increasing revenue or reducing costs. Now, these technologies are accessible to all, including teenagers and younger generations, sparking widespread interest and opening up new market opportunities. And with this comes a responsibility to not only teach the younger generation to use (artificial intelligence) AI but also, critically, to critically evaluate its outcomes.
In this episode, we're joined by Dr. Eva-Marie Muller-Stuler, a data analytics and AI pioneer who has garnered global recognition for her expertise in AI governance for over two decades. She's a sought-after advisor to governments and international institutions such as the UN, UNESCO and ICGA. Dr. Eva-Marie Muller-Stuler has been honored as one of the Top Ten Women in Data and Analytics by Analytics Insight and is currently a partner at Ernst and Young Data and Analytics. Her insights on the societal changes AI brings and the technology's risks and rewards may surprise you!
Tune in for an enlightening conversation that bridges the gap between AI technology and human understanding.
I'd rather read the transcript of this conversation please!
Intro - 00:00:03: Data Masters is the go-to place for data enthusiasts. We speak with data leaders from around the world about data, analytics and the emerging technologies and techniques data-savvy organizations are tapping into to gain a competitive advantage. Our experts also share their opinions and perspectives about the hyped and overhyped industry trends we may all be geeking out over. Join the Data Masters Podcast with your host Anthony Deighton, Data Products General Manager at Tamr.
Anthony Deighton - 00:00:39: Welcome to another episode of Data Masters. Today's guest is Dr. Eva Maria Muller-Stuler, partner at Ernst & Young Data and Analytics, based in Dubai and recognized by Analytics Insight as one of the top 10 women in data and analytics. Before becoming a partner at Ernst & Young, Eva Maria worked for IBM and founded her own company. Laniakea Labs. She's a noted expert on AI governance and over the last 10 years has advised governments and institutions on the topic of responsible AI. Welcome Eva Maria.
Dr. Eva-Marie Muller-Stuler - 00:01:17: Hello, great to meet you Anthony.
Anthony Deighton - 00:01:19: You've had a remarkable career in technology and data and analytics. So I'm sure all of our listeners would love to know a bit more about your background and the stops you've made along the way and how you landed as a partner at Ernst & Young.
Dr. Eva-Marie Muller-Stuler - 00:01:34: Absolutely. So I studied actually at university, mathematics is my main subject and a minor in computer science and in business. 20 years ago, much longer than 20 years ago, 25-30 years ago, that was very, very unusual. Everybody was asking me, so why are you studying mathematics? Do you want to become a teacher? There are no jobs for mathematicians and how odd to compute, combine it with computer science and definitely why is business there? And then started my career in computer restructuring valuation, where we were building financial models to understand the impact of restructuring processes and so on, on the balance sheets. And that has still up to today formed the way that I see data science and AI. For me, it's the two buckets to it. One of them is research, and the other one is corporate performance improvement. Does it help you to make better, faster, cheaper business decisions when it's corporate performance improvement? Anything else should be classified as research. I did my PhD in Machine Learning Algorithms in the Medical Field. And after that, I went over to live in London and we started probably one of Europe's first data science and AI teams at KPMG in Business Consulting. Everyone was always asking us again, why business consulting? Is it not a technology's job that we're doing? And for us, the focus was very clear. No, we want to help businesses make better decisions. And that's what we're using the data for. And it's not that we're using technology to find something or data to find something. We are very clear on what the business is doing and how we can support it. It was the beautiful wild, wild west before any data protection laws. We were able to build massive connected data systems. We were able to get the phone signals from everyone, every phone call they made. And this also led us, especially me and my team, to realize how much power we actually have. We had a long time when we were joking and saying, why are we building AI solutions for the data? If you can just quickly blackmail people by just filtering what we find in their data. And that's how I started working with the UK government and with other governments around the world. I was saying here, There is something happening in that field. We have to be aware of the changes that we'll be facing as a society, but there's also a lot of risk in what we're doing. There's always the upside and the downside. In 2017, IBM called me and said, we're looking for someone to build the Region. So I moved to the Middle East. I'm also CTO for Artificial Intelligence, here at IBM, and we were really... putting data and data science on the IBM agenda. We were working on setting up the data science elite team. I was running the Center of Excellence for Data Science. And then a year and a half ago, I joined Ernst & Young, also here in the Region, to really build their data science practice.
Anthony Deighton - 00:04:22: So in that sense, I think we actually share a bit of background since my undergraduate degree was in mathematics and economics, to be fair. So I think this idea of connecting business value to data and analytics and now to artificial intelligence, clearly a hot area and also, to be fair, one that's grown significantly in the last 10 to 15, 20 years as we've come out of our education and into the workforce.
Dr. Eva-Marie Muller-Stuler - 00:04:48: Absolutely.
Anthony Deighton - 00:04:49: So you've really been at the forefront of how artificial intelligence and machine learning has evolved over the last 10, 15, 20 years. More recently, there has been a tremendous amount of hype around generative AI, MLOps, and other areas. So a question I've been asking a lot of guests these days, ChatGPTs, Generative Transformer Models, is it overhyped or underhyped in your view?
Dr. Eva-Marie Muller-Stuler - 00:05:15: Both. I think it is a very interesting tool, technology and algorithms. We are at an interesting tipping point where we have far more processing powers. We have far more data that we can use and we can use far more powerful algorithms to analyze them, to find patterns. At the moment, they're still not robust. They're still giving a lot of false answers. And I think what is happening now is a lot of people are starting to be able to play with data science and AI. In the past, I think most people didn't realize to what extent Google Maps is actually also applied data science in AI. But now it gives them the vowel effect. It gave them the thinking of... There are actually things that are possible that humans can't do. I absolutely think something like Google Maps and finding the optimal way is something that humans can't do to that extent. But being able to interact, to find language and so on has gone to, it has basically lifted it to a higher level, on that. Now, pretty much every company we deal with is starting to wonder how they can use it in their processes and how they can implement it. And that's where I'm saying it's also overhead because the costs are significant, the risk is significant, and it doesn't stop you from evaluating the answer. So it takes a lot of time to train on your Personal Data or on your Company Data. So there are a lot of thoughts we have to think about and say, how can we actually bring that into our clients?
Anthony Deighton - 00:06:45: So one thing I've often talked about with regard to these generative models is that the most disruptive thing that OpenAI did is the Chat part of ChatGPT, which is to say that it took this technology and gave it a consumer interface that allowed, to your point, lots of people to interact with it and recognize the... power that it has, but also some of the risks that it represents. Is that a fair way to think about what you just said?
Dr. Eva-Marie Muller-Stuler - 00:07:12: Yeah, it made it accessible. It made it usable for anybody who wants to play around with it. Same what we see in all the image recognition, generative AI models. Suddenly everybody can take two pictures and say, build something new or build me something that this and this and this represents, and it made it mainstream. And it was really boring. Before, I thought data science and AI was a little bit boring. It wasn't fun. It had serious applications. It was what can we find in our data and what can we use it to make more revenue? or to cut costs. And now it's something that every teenager can play with. And that brings a lot of interest in the market. And that's also why the wrist side becomes so interesting because you can train teenagers and younger generations to interact with it. But also we realize we have to train them to actually question the outcome.
Anthony Deighton - 00:08:06: So I was going to ask you about that because you've worked, as you indicated, as an advisor to governments and International Bodies, even the UN, on the risks that AI represents. I loved your sort of introductory story, this idea that one business model for artificial intelligence could be blackmail, probably not the most ethical of which you might pick. But in any case, how do you think about some of these risks from a government perspective? How did the... these large government agencies, whether it be the UN or even national governments or even local governments, how do they think about some of these challenges that they're trying to overcome?
Dr. Eva-Marie Muller-Stuler - 00:08:44: There's this beautiful saying that basically says, to make mistakes as human, but to really mess things up, you need a computer. With a computer, we are able to make so many more decisions per millisecond that a human couldn't do. When it comes, for example, to image recognition and things like that. And we know that our world is biased. There's no doubt about it. And so we always start building these models on biased datasets. I actually disagree with... popular opinion that the biggest risk of data science and AI is the point of singularity, that they're taking over, that they're cutting us out of the world. I think the biggest risk that we see at the moment is that we have to ask ourselves what is the kind of world we want to live in. How can we assure that these models are fair, that they're transparent, that they're safe to use, that they're accurate? that they're transparent and audible and that they actually protect our security. That you cannot ask for personal information on ChatGPT about somebody and it just gives the answer about things you maybe shouldn't know about that person. So I think that the risk is, especially with the biased data, well, that we see a lot of models coming out of the US, and especially East Coast and West Coast. We take these models then and apply them, for example, in health care, here in the Middle East. I guarantee you there is very little representation of Women over 65, or Emiratis, in these datasets. Yet we take them and we take their answers as knowledge without really asking how they were trained. And these are the risks that I see for our future, that we are underestimating the effort and that it costs to build accurate models. When we look at... all of data science and AI and meaning as simple as there's data, we do anything with it. It could be a linear regression. It could be a large language model. It could just be slicing and dicing, and an outcome. I actually believe of the dashboard that we look at, 99% of them are And they're wrong because the data was handled the wrong way. The data didn't exist. It might be the wrong algorithm. It might be overfitted, or they're just simply Yeah, presented in the wrong way that the decision maker reads it the wrong way and makes a wrong decision based on it. And that can have all sorts of risks from financial, legal, reputational, any kind of risk that is out there. We can increase using data science and AI. It's two sides of a coin. We can make it better or worse.
Anthony Deighton - 00:11:16: Yeah, and I think this idea that automating things simply increases the pace with which we make bad decisions or make incorrect or make biased decisions. So the risks associated with automation is really a risk of runaway systems. We see this in trading. We see this in algorithmic training, for example. I really like this idea of ethics in AI, and because in some ways it's an oxymoron, right? By its nature, AI is a system. It's not ethical. It's just a computer. Computers, by their nature, don't have ethics. Clearly, people do, systems do, rules do. So how do you think about ethics in the context of AI?
Dr. Eva-Marie Muller-Stuler - 00:11:57: I love that point because it really, a lot of, when we look at, for example, the IEEE standards and so on. A lot of the concepts are saying we need to train people how to think ethically and ask ethical questions and get an understanding of where the risk could be. I often don't think that the solutions were built badly. And I actually make a difference between ethical AI and responsible AI. Ethical AI for me is asking the question ofAre we doing the right things?And responsible AI, trusted AI is, how do we do them right? and we have a big gap, in these conversations because you go to the government, or the working group, and where you have lawyers, you have politicians, you have ethical researchers, and you need all of them, but you always have incredible under-representation of the technical community, they don't come enough to these meetings. Which often ends up with, we have all these amazing buzzwords, but we lack the real understanding on... How do we actually implement that? I led a large study at IBM on explainable AI, in the first question where we argued weeks about it: what does explainable actually mean? And explainable to who? Is it explainable to Anthony when he's asking why I did not get a seat on the Titanic lifeboat? That we can say, oh, because of ABC? Has Anthony arrived to ask, how does a model make the decision? Which are completely different questions to start with. Or does a person who's actually saying you go on, you go off, that he or she needs to know how the model works and what the downsides are. Understand that these models are probabilistic. So it's not really 100% for sure that Anthony should be or should not be on the lifeboat. But we use AI and data science more and more for high-stake decisions. And with that, we really increase the risk we bring into our society.
Anthony Deighton - 00:13:52: So I love that. And a lot of times, if we leave humans in the context of these decisions, they may make ethical decisions, but they also are by their nature random. And if you ask a person, why did you make this decision, their capacity for lack of a better term, explaining themselves, I feel like I ask my kids this all the time, explain yourself, they are very poor at answering that question. Adults and children both have a hard time. So it's a lot to ask a system to explain itself, especially when the response could be probabilistic by its nature.
Dr. Eva-Marie Muller-Stuler - 00:14:24: Yeah, absolutely. We also face another problem that we call the Colin-Rich Dilemma. And that is, when we implement or when we build the technology, we often don't anticipate the impact it will have on society. The moment we see the impact, it's too late to react. So we saw that with the development of Computers, where everyone was like, who needs this? There might be a demand for four Computers around, personal Computers around the Whole World. With mobile phones, I remember everyone going, I don't want to be reachable all the time. I will never in my whole life buy a mobile phone. And even with apps like Tinder and so on, now that we see the impact of a like button on Facebook, it is too late to actually say how we react to it. We can't just switch off the internet because it's so ingrained in our way of living already, and asking these questions, when they are developed, is nearly impossible to answer because we still don't really understand how it will be used. Nobody more than we use the internet for watching cat and dog videos.
Anthony Deighton - 00:15:25: Right. And hopefully it can have a bigger impact on society than that. Although the idea of entertainment as a primary use case for, it may be said more fairly communication, primary use case for a lot of these technologies itself. Interesting. I wanted to return to something you'd said a while ago, which was, if I could paraphrase what data you feed into these systems for the purpose of training them is inherently biased. And you made a point that I make all the time, which is that most people's dashboards and analytics are woefully out of date, incorrectly treating data in silos, you know, looking at incomplete sets of data and the underlying concept there is one of truth. The idea behind a dashboard is that it represents in a truthful way, some metric or dimension of the business. And I think we're seeing this same criticism in AI in particular around these Generative Transformer Models, is that they have trouble telling the truth. And worse than that, and maybe it's not worse than that, actually, as I say it out loud, when I put a number on a dashboard and I say the margin is X, margin is 3%, the essence of that dashboard is that it's authoritative. The margin is in fact 3%. That's also true of these transformer models in that they want to create a narrative that seems authoritative. They're being trained to speak and with authority. Even when the underlying facts are wildly inaccurate. And so you make this great point about the distinction between responsible and ethical AI. Is that also fair to draw a relationship between truth and ethics in AI? Or is that really the same idea?
Dr. Eva-Marie Muller-Stuler - 00:17:07: Absolutely, yeah. So the accuracy of models is one of the main pillars of when we talk about trusted AI or ethical AI. The robustness, data privacy protection, the bias. And Echo See is one of them, and transparency. So transparency means You know that for example the decision was made by a model, and you also know what purpose the model was built for. So with something like ChatGPT, it needs to be very clear that this is built for experimenting, that there is a big caveat that is not 100% true. Don't copy and paste the homework that you ask it to write, and put it into your report because there is a... very good chance of you getting caught. Once your teacher is trained on how to spot it, it's actually very easy to spot because you see numbers that are not verified, and it doesn't understand the context of where the numbers come from. So just because they're in the right order, it might sound like, okay, here's the balance sheet, here's revenue, and here's the 20%. And there's somewhere the next word is gross margin. So we take that and we present that as the real gross margin. There was actually a sentence before saying, when it was adjusted because of this and this and this, or it was a gross margin only for the subject. Mag drop it! But being presented in an authoritative way... We need to learn not to trust them. We need to learn how to challenge them. It is actually also one of the things during COVID. Our data collection was our main issue during COVID. We had one doctor testing every person who came in. And we had other doctors with everybody who came in saying, Yeah, go home and stay away from everybody. And then we had big red hotspots and green areas. And the green areas were probably big red hotspots as well. There were just no tests. And that data collection issue we have across the whole bench. And if we don't have a basis of truth and we don't understand how to generate the basis of truth, there's nothing we can do to get the outcome that is actually truthful and insightful.
Anthony Deighton - 00:19:20: So would it be fair to conclude that the next five to 10 years in data and analytics will be much more focused on the core data, like finding truth in the underlying data, fixing data mistakes, data errors, data collection bias, etc. Versus or instead of how we present the data to users through dashboards and analytics, not to say that that's not important, but largely that problem has been solved. And the real question is, what do we feed into these systems, either from a modeling perspective or from a raw data perspective?
Dr. Eva-Marie Muller-Stuler - 00:19:54: Yes, I think that the focus on the data is already increasing. You see that? In the past, we always thought a great data science project was a boy in a hoodie who can code and has the fanciest algorithm, and He or she didn't know anything about the business at all. Just really, really smart, coolest algorithm ever. And then nothing was questioned anymore. And we saw a lot of companies investing a lot of money into their data science and their IT teams, but you're not getting any value out of it. The understanding that a data science team actually needs to be a profit center, not a cost center, is still often not there with companies. They're running models that are far too complicated on data that doesn't really exist and build amazing dashboards and say, okay, this is the truth. So yes, I agree that we really need to focus on How do we get the right data for our questions? And how do we make sure our data set is of a quality that is good enough to ask the question and model the question. And then I'd say most of our questions today that we are asking in business can probably still be best solved by good data engineering and then a linear regression.
Anthony Deighton - 00:21:11: Yes, it's shocking to me how many times we always come back to ordinary least squares as the best answer to almost every problem. And sometimes I think it's a reasonable point to sort of think about this idea that sometimes simpler is better. And this idea that we need more complexity by its nature is the best answer to the question. As we sort of wrap up, I wanted to shift to a completely different topic. You said something under your breath there that I want to key on for a second, which is that for many data scientists, our perception of a data scientist is a young man in a hoodie. And I think that is a bias that people have. They have this imagination of what a data scientist, who a data scientist is, what they look like and what gender they are. And clearly you've been incredibly successful in this field. You were recognized as a top 10 most influential women in technology. What advice would you give to someone who doesn't fit that mold? And I'm not referring necessarily to their clothing choices. But if you were, if someone was an aspiring young woman that was just graduating, entering the workforce and they had an interest in technology and maybe even an interest in data and analytics. How do you think about, what advice do you give them to help them be as successful as you've been?
Dr. Eva-Marie Muller-Stuler - 00:22:27: I'm laughing with a hurting heart if I'm really honest because you can't imagine the amount of times people said to me as a compliment, oh you don't look like a data scientist. This is not a compliment that shows how biased and misogynistic your worldview is, and The only reaction to that is like, and you don't look like a misogynist, but here we are. And we don't see the brains from people on the outside. So for me and the comments I got on, Oh Eva, maybe you should wear, less high heels or you should wear glasses or maybe you should turn up in a hoodie and comments like that. We are in a world where we have to learn to accept our diversity. We have to learn that... It doesn't matter what you look like, you can be top of the game technically in your field. For me personally I'd always say I know my core strength, I know what I really love and I really love mathematics. And I know that when I go into the meetings I'm top prepared and I'm normally the one who knows this subject better. Stay true to yourself and try to not get intimidated by... somebody who might say the most ridiculous things, but in the most confident, loud voice possible. Normally he might not be right, and learn that it took me a while myself, and saying, no, I'm actually here the expert in the field. And what he's saying is actually not working. I think that was for me, the confidence of really believing that I know what I know, was a big step forward in my career.
Anthony Deighton - 00:24:01: And to add to that and to return to a word you used earlier, which is this idea of diversity, if we think about the quality of models, the quality of models increases as the diversity of the data input into that model increases. And I think that's naturally true of human tasks as well, of decision-making. So if we can get more diversity-
Dr. Eva-Marie Muller-Stuler - 00:24:23: The problem with the mathematical formula is that the error of a crowd decreases with their diversity. Because especially when it comes to data science somebody might say hold on, where are the Emirati women? Where are the Indian women? Where are the older generation? Which the 21-year-old might actually not be aware of, that they're all missing.
Anthony Deighton - 00:24:43: Yeah, so many organizations would do well to be biased against hoodies in their interviewing process as a mechanism of just simply creating more diversity of opinion.
Dr. Eva-Marie Muller-Stuler - 00:24:52: Yeah, absolutely. Yeah.
Anthony Deighton - 00:24:54: Well, Eva Maria, it's been a pleasure, and I appreciate you making the time and joining us today. And thank you for being on Data Masters.
Dr. Eva-Marie Muller-Stuler - 00:25:01: Thank you. Thank you so much, Anthony. I really enjoyed it.
Outro - 00:25:05:Data Masters is brought to you by Tamr, the leader in data products. Visit tamr.com to learn how Tamr helps data teams quickly improve the quality and accuracy of their customer and company data. Be sure to click subscribe so you don't miss any future episodes. On behalf of the team here at Tamr, thanks for listening.