Michael Stonebraker and the Founding of Tamr

Who is Michael Stonebraker?
Dr. Michael Stonebraker is a pioneering computer scientist for more than 50 years and entrepreneur renowned for his groundbreaking work in database technology. With a career spanning several decades, Stonebraker has made significant contributions to the field of database management systems (DBMS). His innovations have led to the development of some of the most influential database systems, which have shaped the way data is stored, retrieved, and managed across industries. He was the main architect of the INGRES relational DBMS; the object-relational DBMS POSTGRES; and the federated data system Mariposa.Dr. Stonebraker has received numerous accolades for his work, including the prestigious Turing Award in 2014, often referred to as the "Nobel Prize of Computing," recognizing his fundamental contributions to the concepts and practices underlying modern database systems as well as their practical application through nine start-up companies that he has founded. His research has continually pushed the boundaries of data management, making him a central figure in the evolution of modern database systems.
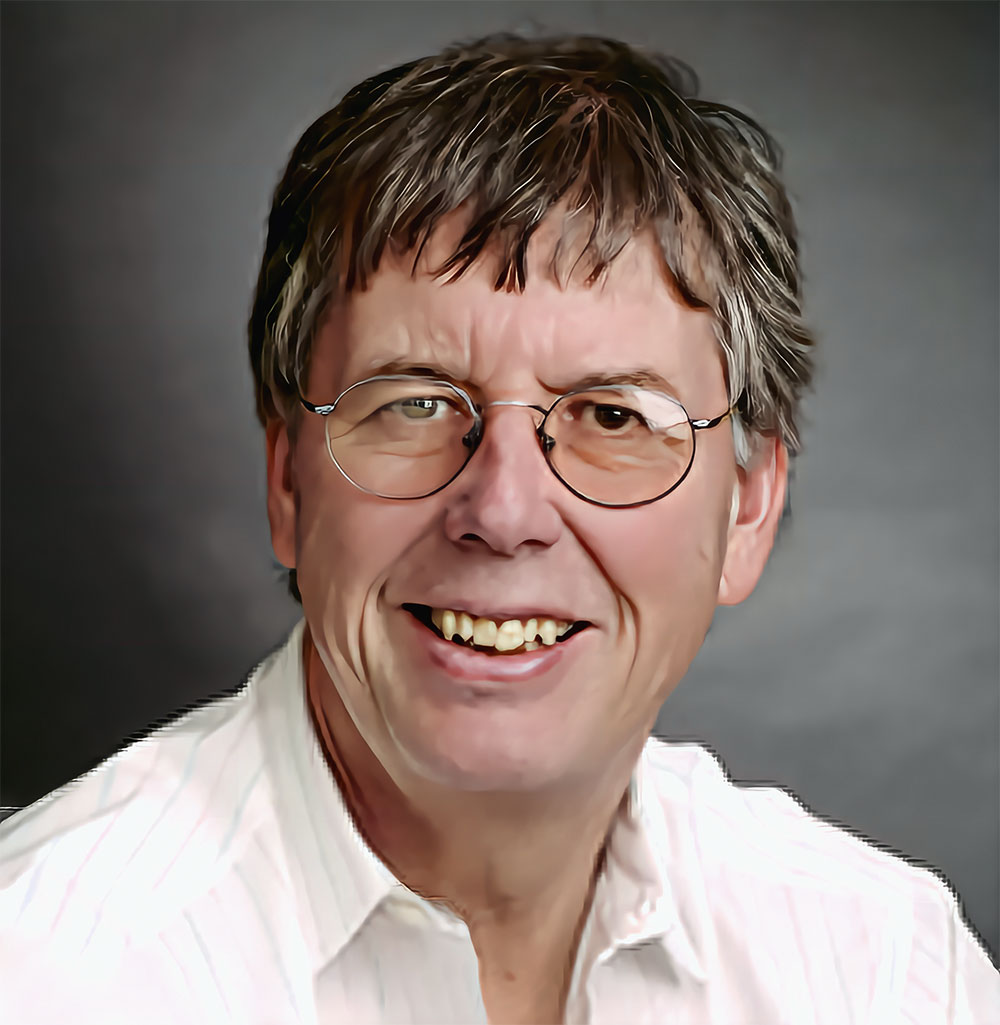

The Birth of Tamr
In 2013, Michael Stonebraker, alongside Andy Palmer, co-founded Tamr, a company dedicated to addressing one of the most significant challenges in data management: data mastering and entity resolution with human-guided machine learning. Tamr was born out of the recognition that traditional approaches to integrating and unifying large datasets were not keeping pace with the explosive growth of data in organizations like Western Union, Toyota, Old Mutual and Santander.
Tamr's Vision and Innovation
Tamr's mission is to accelerate the digital journey of every business by enabling continuously updated and consumable clean data. Tamr develops data products that use battle-tested AI to speed the discovery, enrichment and maintenance of the golden records businesses need to accelerate growth. Tamr’s AI-powered, human-refined approach delivers value in days, not months or years all while lowering project and operational costs when compared to MDM or DIY solutions. By connecting data across source systems and incorporating 1-click, 3rd party data enrichment, Tamr delivers accurate, comprehensive and durable data ready for consumption.
17 Patents and Counting!
Our rich history of innovation and excellence
Innovation was at the heart of Tamr’s founding at MIT, and it remains a core part of our DNA and company culture. As new standards in scale and efficiency evolve, Tamr continues to lead the market by delivering state-of-the-art technologies that meet the needs of the modern data ecosystem. Our patent portfolio underscores Tamr’s commitment to providing innovation to businesses looking to accelerate their success using accurate insights fueled by clean data.
Tamr’s pioneering, patented technologies help customers:
- Provide automatic, reliable survivorship at scale, enabling the automated creation and maintenance of Tamr IDs
- Create a novel, yet straightforward, method of estimating overall accuracy given a very small amount of human input
- Combine machine learning with human oversight to develop an innovative system that accurately curates data at scale while delivering on the promise of cost effectiveness
- Pioneer an approach that seamlessly integrates manual data curation into a versioned data product
- Promote the reusability of human feedback across multiple source data
- Capture user feedback within the context of the application they are using, making it easier for curators to view the feedback in context and fix it
- Translate user feedback into the input that machine learning models require so that the training remains unbiased, stable, and durable, even when the data or model changes
- Enable the machine to quickly focus on comparable records, making it easier to deduplicate data at scale
- Use data multiple times in multiple ways, dramatically reducing the amount of training required to achieve a high level of accuracy
- Enable the machine to quickly focus on meaningful categories when applying the model to find a best-match category for a given record
- Scale to very large feature datasets by providing alternatives for the use of geospatial databases, even between disparate feature types such as point of interest and building footprint, while avoiding accuracy trade-offs due to projection
- Translate the technical needs of the machine learning active learning system into practical questions that a data expert can answer