How is Tamr Different from MDM and ETL Tools?
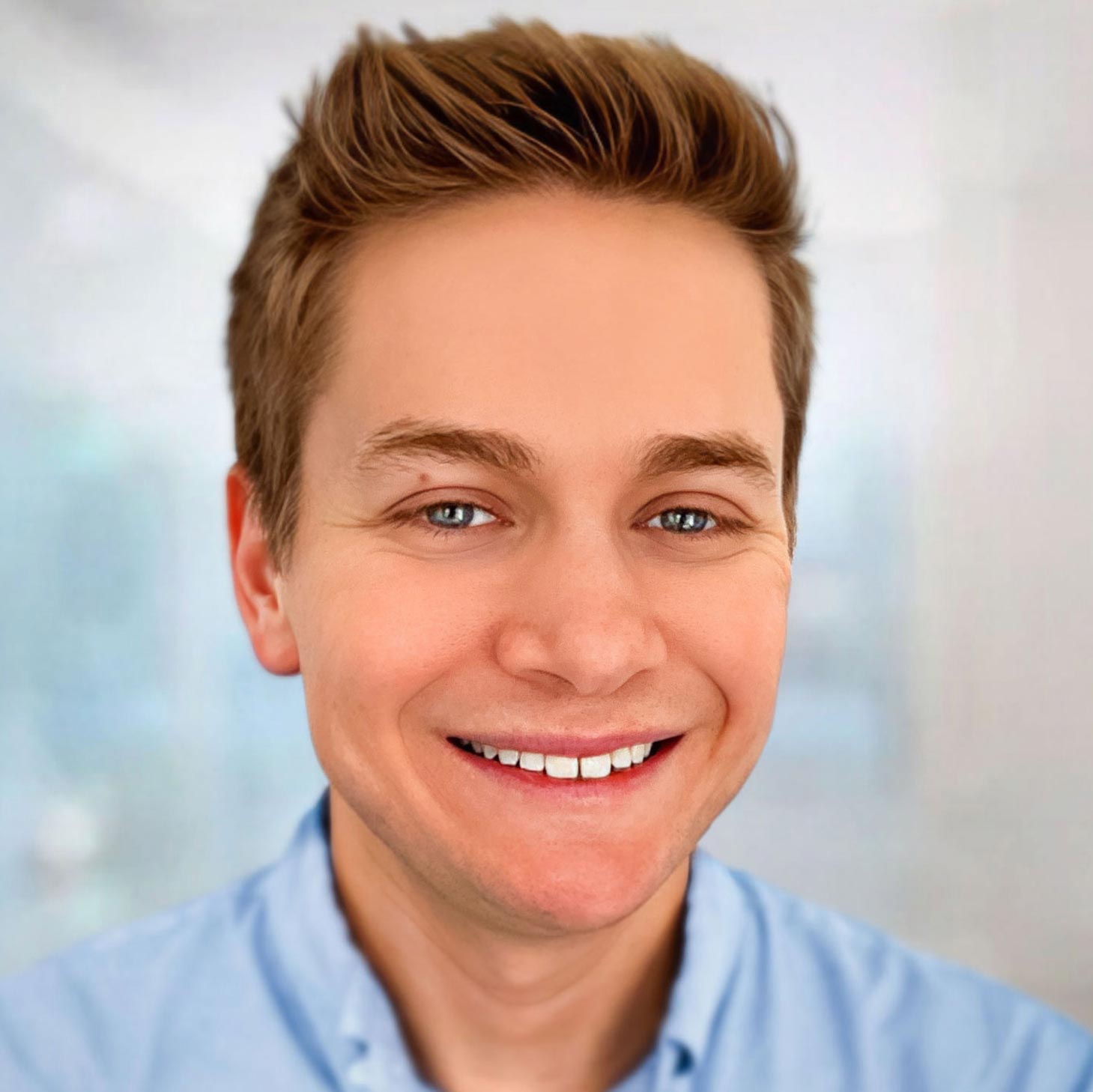
There is a lot in flux with the data management industry, and naturally, it’s causing confusion. Companies collect data at a rapidly expanding rate, and the old processes in place to master this data severely limit their ability to make sense of it all.
As we know, unmastered data leads to a host of problems from the inability to optimize business operations effectively to leaving you susceptible to data breaches and compliance issues.
In this blog post we review the key features of Master Data Management (MDM) solutions, compared with Extract, Transform, and Load (ETL) solutions, and discuss the benefits of Tamr cloud-native data mastering, ML-based approach for data mastering is compared to ETL and MDM.
MDM vs. ETL
What are your current data mastering options and what are the pros and cons of each? Let’s review:
Master Data Management (MDM): The MDM process involves creating a master record where all entities used across the organization are defined. The idea is to merge all the individual records and match that to the entity in the master record using rules to accomplish the task. Here’s a few examples of what this looks like from Michael Stonebraker’s 7 Tenets Of Scalable Data Unification:
- “Dick” matches “Richard” in the name field
- -99 matches null in the salary field
- If systems A and B have different values for address, then use the one from system A
MDM Pros: MDM provides a “golden record”, which is meant to be the “source of truth” about an entity that other sources can reference downstream. These systems also provide insight into the lineage of these records and flexibility in defining how these records are created. In theory, when done without errors, you should have no issue with duplicates or unmatched data, and be able to provide consumption tools with an accurate view of each entity.
MDM Cons: You’re relying on a rules-based golden record, which requires a human-intensive process to deliver. This is not scalable and is dependent upon continuous, manual review of exceptions. Because of this, you will not only leave a large portion of your data unmastered, but you also pay a premium in resource costs to leave yourself susceptible to human error.
Extract, Transform, & Load (ETL): The ETL method is a widely adopted data mastering process that’s been around for 20+ years. It involves creating a global schema up front using a programmer to understand how the schema is used and writing conversion routines, cleaning and transformation routines, and continuously updating it over time to ensure accuracy.
ETL Pros: ETL is a very effective way to move data, and can also be used to perform mastering when simple rules will suffice (e.g., “Inc.” equals “Incorporated”).
ETL Cons: It’s impossible to scale using ETL since it’s so laborious in nature. This is not a viable option for companies that collect a large (and continuously growing) amount of data. ETL is also not designed to deliver a golden record, a key output of the mastering process that is necessary to drive consistency across consumption points.
It’s hard to believe that MDM and ETL are the most widely used data mastering processes given their inability to scale. For years, companies have had to settle for not being able to leverage a large portion of their data until now.
The Modern, Agile Solution to Data Mastering
Having a platform that allows for complete data unification is the solution to data mastering at scale. It works by taking your data from all facets of the business and unifying it by using a combination of machine learning and human expertise. It’s the solution to MDM and ETL shortcomings, allowing companies to master all of their data in an efficient and effective manner.
Some platforms (like Tamr) even use machine learning and automation to continually evolve and update as your data changes and grows.
Just how big of an impact can a data mastering platform like Tamr having on your bottom line? For tech giant GE, it meant a savings of 80 million.
The Advantage of Cloud-Native Master Data Management by Tamr
Tamr differs from traditional data tools like MDM and ETL by using an agile approach to tackle data mastering, as well as leveraging the scalability of a cloud-native data mastering implementation. Learn more how Tamr’s approach is different than traditional MDMs, like Informatica.
As we all know, agile completely transformed software development, and it’s set to do the same with data thanks to Tamr.
Bridging the Gap Between Data and Analytics
Tamr connects internal and external datasets (including datasets from various CRM and ERP systems, external reference data aggregators and third-party datasets). It uses proprietary machine learning technology to produce higher quality, up-to-date, curated datasets for downstream analytics programs. Tamr’s output is clean, consolidated data that can then be used to power visualization tools such as PowerBI, Qlik, Tableau, and Thoughtspot. In addition, Tamr’s technology engages data experts effectively through simple yes/no questions to provide feedback on data outliers and train the ML models to meet unique business needs, driving higher data accuracy and bridging the gap between data and analytic outcomes.
Get a free, no-obligation 30-minute demo of Tamr.
Discover how our AI-native MDM solution can help you master your data with ease!