How to Tackle Volume, Velocity and Variety in Clinical Data Management
The digital transformation towards more agile, data-driven operations for clinical studies have been a critical part of every life science company’s roadmap as they build towards reducing the time and costs of drug development in order to get medical products approved and out in the market to patients faster.
However, while every organization’s exact data priorities are different, initiatives to implement a game-changing clinical data warehouse, translational data warehouse, statistic computing warehouse, or “the ultimate R&D data hub” have proved challenging due to a large variety of documented reasons. For some organizations, by the time a 2-5 year data warehouse is built, the needs have changed and R&D users have moved on to their own preferred approaches and tools more convenient for interacting with their specific datasets – even if the approach is less efficient or more manual.
This “siloed approach” to clinical data management is not scalable nor sustainable towards building a world-class life science R&D organization. The recent popularization of FAIR practices, also requires there be a more robust approach to tackling the big clinical data problem where the underpinning challenges are around data volume, velocity, and variety. If the organization is able to make available all their clinical data in one unified format for analysis, there is tremendous opportunity to change how clinical research and operations are done.
Working with data-driven life science companies like GSK and Amgen, Tamr has developed clinical data conversion solutions specifically targeting the data challenges in data volume, velocity, and variety. One of the most obvious places to look for data volume and variety is the attic of legacy clinical study data that many life science companies have kept over the decades, where the different study data capture methods, standards, and content variety make it impossible, or too expensive, to analyze.
Whether it is to standardize this treasure trove of clinical data into a CDISC standard such as SDTM, ADaM, BRIDG, or a completely custom schema model, Tamr have repeatedly delivered transformational results by accelerating the data harmonization process with robust, scalable data pipelines that can process over 10 billion records / day and a machine-learning approach that can accelerate the schema mapping and transformations of 200 clinical studies within a year, with only two resources (and in another case, over a 1000 studies in less than 2 years).
Tamr’s approach to clinical data conversion for legacy studies
There are several areas where an organization can implement the Tamr clinical data conversion solution. Among our customers, the application of Tamr to harmonize legacy study data into a unified schema such as CDISC relies on installing a Tamr instance into a data flow similar to the one depicted below.

Tamr can typically be installed on any on-prem or cloud server as long as it has access to the study data. In most cases, life science customers have either chosen to export SAS files directly into Tamr for ingestion, or have Tamr pull from a relational database to begin data conversion processing.
Within Tamr’s UI or API interface, a clinical data expert can map some data attributes to a configurable schema for each CDISC domain, for example, and Tamr will then profile, learn, and offer probabilistic suggestions on other attributes that may map into the same “unified schema attribute”.
For example, in the table below, Tamr is able to suggest with 99% confidence that an attribute innocuously labeled A932SID from the dataset GN-932-AE maps to USUBJID due to commonalities in name and values among the attributes that have already been mapped. Suggestions like these, when automated via API at scale, significantly reduce human-error and manual efforts, if there are tens of thousands of attributes to review and map.

Based on known documented mapping specifications, the clinical data export can leverage Tamr’s auto-mapping tool to read-in pseudo-code specifications into Tamr transformation code to perform that action. In addition, the data expert can also write and edit Tamr’s SQL-like transformations to decode data records (look-up values), reshape tables (transpose datasets), and transform individual attributes (record transformations and standardization) to complete the data conversion process into a schema such as SDTM.
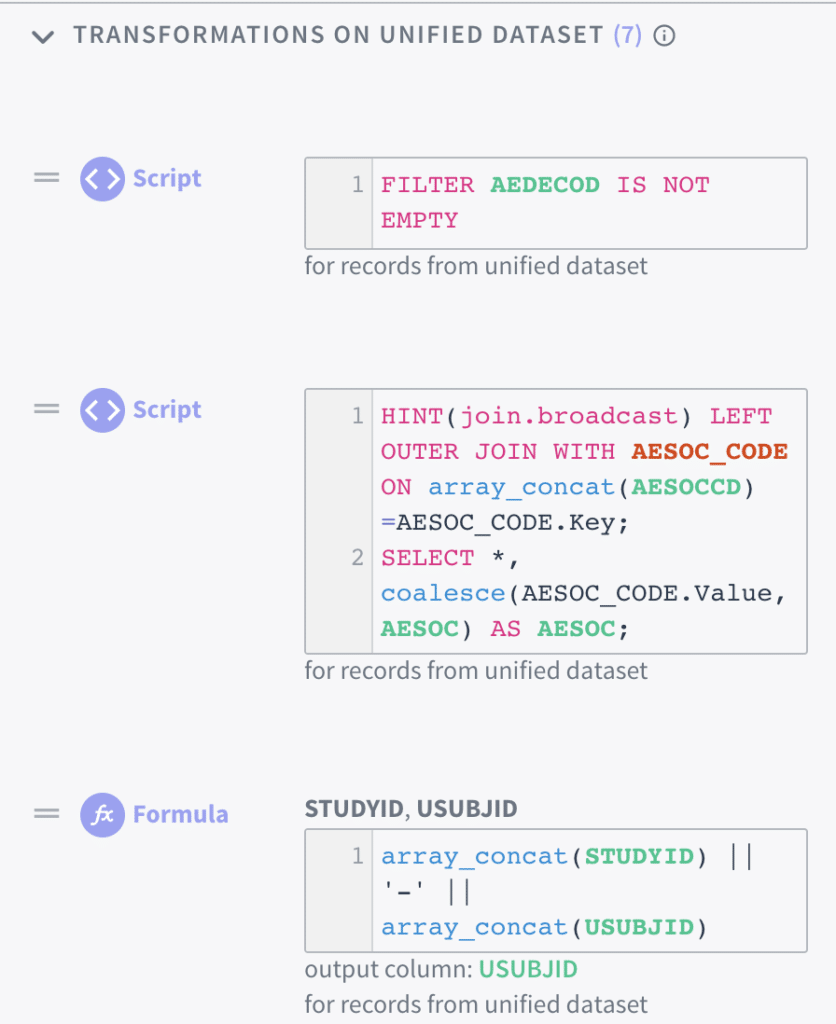
For a more detailed dive into the features of Tamr’s clinical data conversion solution, read the solution paper here.
Conclusion
Life science companies use Tamr to tackle their problems in data volume, velocity, and variety with an agile approach, assisted by machine learning, to realize opportunities promised by the modern mandate for digital transformation.
With the ability to typically show production-level results within 6 months of implementation, Tamr users in life sciences leverage Tamr to provide quick, tangible wins for data-driven clinical operation improvements and analytic insight. I will highlight some examples of the insights our customer have uncovered in another post.
Many of our users know that Tamr’s approach is not limited to study data, and along with our other key technology features such as agile data mastering and classification, have already started leveraging Tamr to unify their real world data, ‘omics’ data, customer data, physician/patient data, product data for IDMP, and more.
To learn more about the role of Tamr in tackling the volume, velocity, and variety in clinical data, schedule a demo.
Get a free, no-obligation 30-minute demo of Tamr.
Discover how our AI-native MDM solution can help you master your data with ease!