Data Mesh 101
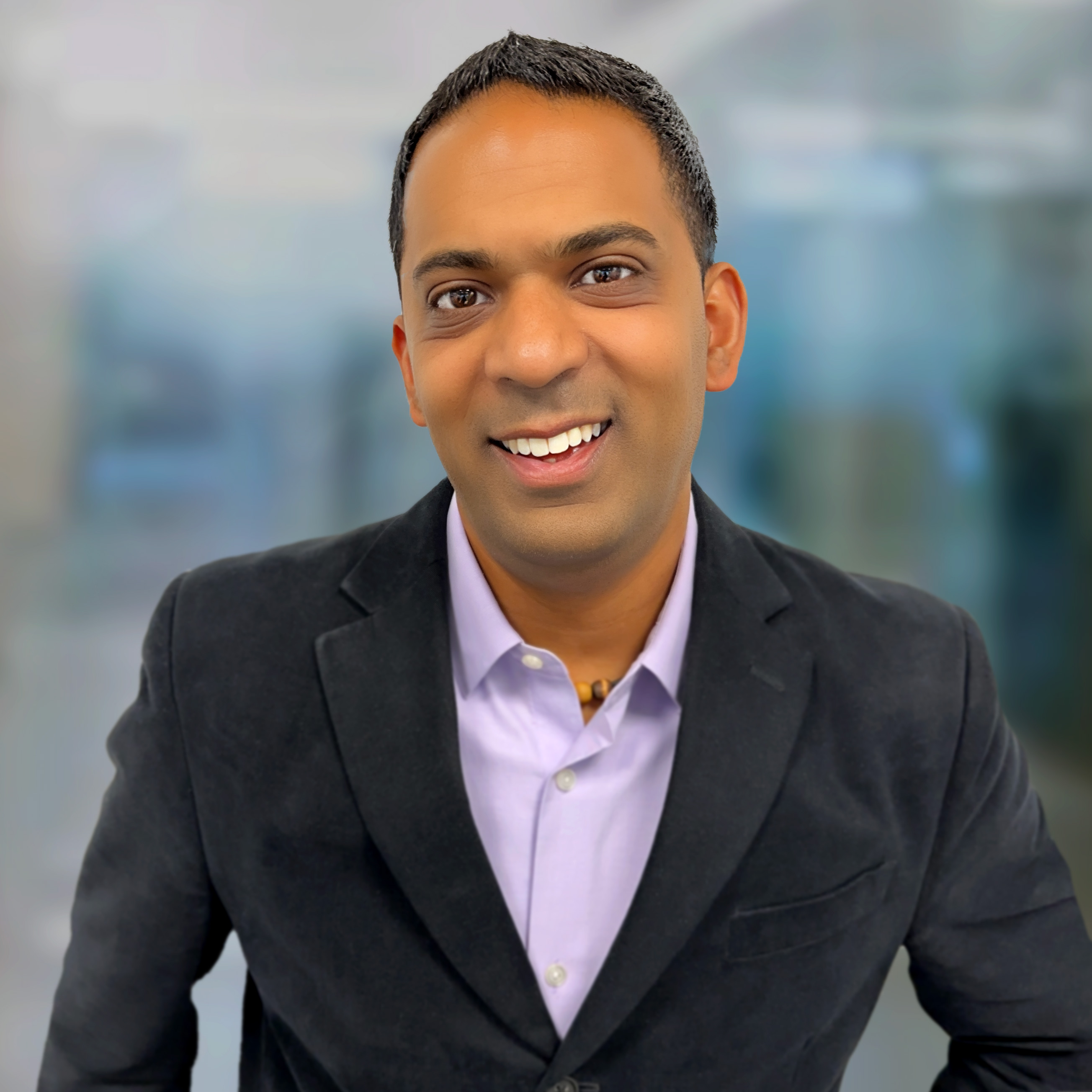
Data mesh continues to grow in popularity in part because data is everywhere. Every system and every process in our personal and professional lives collects and generates data. And the volume and variety of this data is growing at a pace never seen before. To complicate matters further, companies are realizing that the data they capture within their organization is no longer sufficient on its own. Instead, they need to enrich their data with third party sources in order to improve its quality and realize the full potential of their internal data.
As more enterprises consider data mesh as a viable option to solve data challenges, the debate between centralizing and decentralizing data takes center stage. In a data mesh ecosystem, the data, by nature, is distributed and external, and it embraces four key principles:
- Data ownership by domain: bring the data ownership closest to the people who know the data
- Data as a product: avoid silos by making the data teams accountable for sharing the data as a product
- Data available everywhere (self-serve): implement a new generation of automation and platforms to drive this autonomy
- Data governed where it is: introduce a new way of governing the data that avoids introducing risk
But I would argue that it’s not an either/or proposition. Data mesh can support both centralized and decentralized data. But in order to do so, companies will quickly realize that they need a consistent version of the best data across their organization. And the only way to achieve this at scale is through data mastering.
Human-guided, machine learning data mastering is a complement to data mesh. On their own, each produces a good result. But when you put them together, that’s when the magic happens.
See, when you apply human-guided, machine learning data mastering, you clean up your internal and external data sources. You can use data mastering to provide a centralized entity table and persistent universal IDs for users to do distributed queries. And, you can engage in a bi-directional feedback cycle that enables you to clean and curate your data so that you can efficiently and effectively realize the promise of distributed data mesh. It’s a continuous loop – and that’s critical so you can incorporate changes to your data or the sources over time.
Getting Started with Data Mesh
If your organization is considering data mesh as a strategy, there are two things you must do before getting started: understand your data culture and get data mastering right.
Knowing how your organization views data is a critical first step. Do they see data as a value driver or a cost center? If it’s the former, then your data culture is healthy. If it’s the latter, then your first step is to shift the organization’s perspective and prioritize data to drive value.
Getting data mastering right is also a key, foundational step to a data mesh strategy. Too often, I see organizations skip this step, only to discover further down the line that their data is dirty and difficult to trust. Said differently, when you build a strategy like data mesh without investing in your data mastering strategy, it’s like building a fancy new house without ensuring that the foundation is sound. Initially, it may not cause you problems, but long term, you are in trouble.
The reason why these steps are so critical is because data mesh empowers data ownership by a domain owner. But in reality, many domain owners may not want – or be ready – to assume the burden of owning a traditional master data pipeline on their own. Why? Because in a traditional pipeline, it’s difficult for data consumers to get feedback on the data issues being addressed. That’s why data mastering is so critical. With it, you gain functionality that enables you to solicit and capture feedback from those who know the data best.
The good news is that you do not need to start from scratch. Instead, you can start by defining your best practice pipeline and then make this pipeline available to anyone. This baseline pipeline would include best practices such as a standard way to reliably master core entities, correct common data quality issues, and enrich the data with preferred sources. From there, domain owners could tune and modify this asset to address their specific needs.
You can even take it one step further using a persistent identifier such as TamrID. That way, you can create a central, universal key for your primary entities as part of the pipeline. Then, domains are free to use it in a distributed manner for their own purposes.
Data mesh is a journey. It requires organizational readiness (ensuring your culture views data as an asset, not a cost) and a solid foundation (mastering your data at scale) before you even start. And remember, it’s still early days with many yet to take the steps from academic promises into realized outcomes. Organizations that are exploring data mesh see it as an enterprise-level play. But they have a long road ahead before they can declare success.
There’s a lot more to come on this topic, but one thing remains certain: successful data mesh needs human-guided, machine learning-driven data mastering. This is what Tamr provides.
To learn more, download Data Mastering Holds Promise as Underpinning Technology for Data Mesh, a report from 451 Research, a part of S&P Global Market Intelligence, and schedule a demo.
Get a free, no-obligation 30-minute demo of Tamr.
Discover how our AI-native MDM solution can help you master your data with ease!