A Guide to Machine Learning for Data Stewards
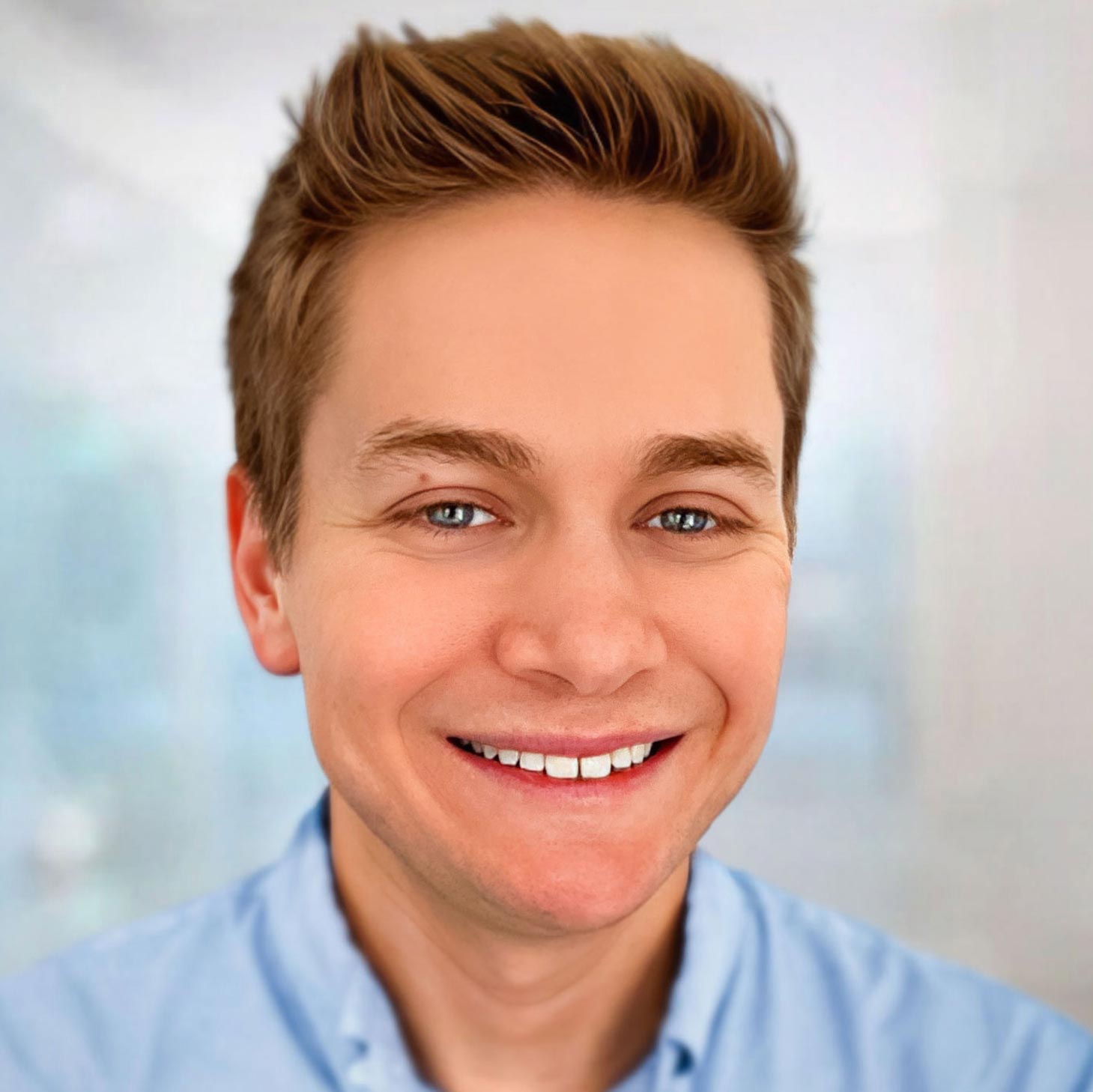
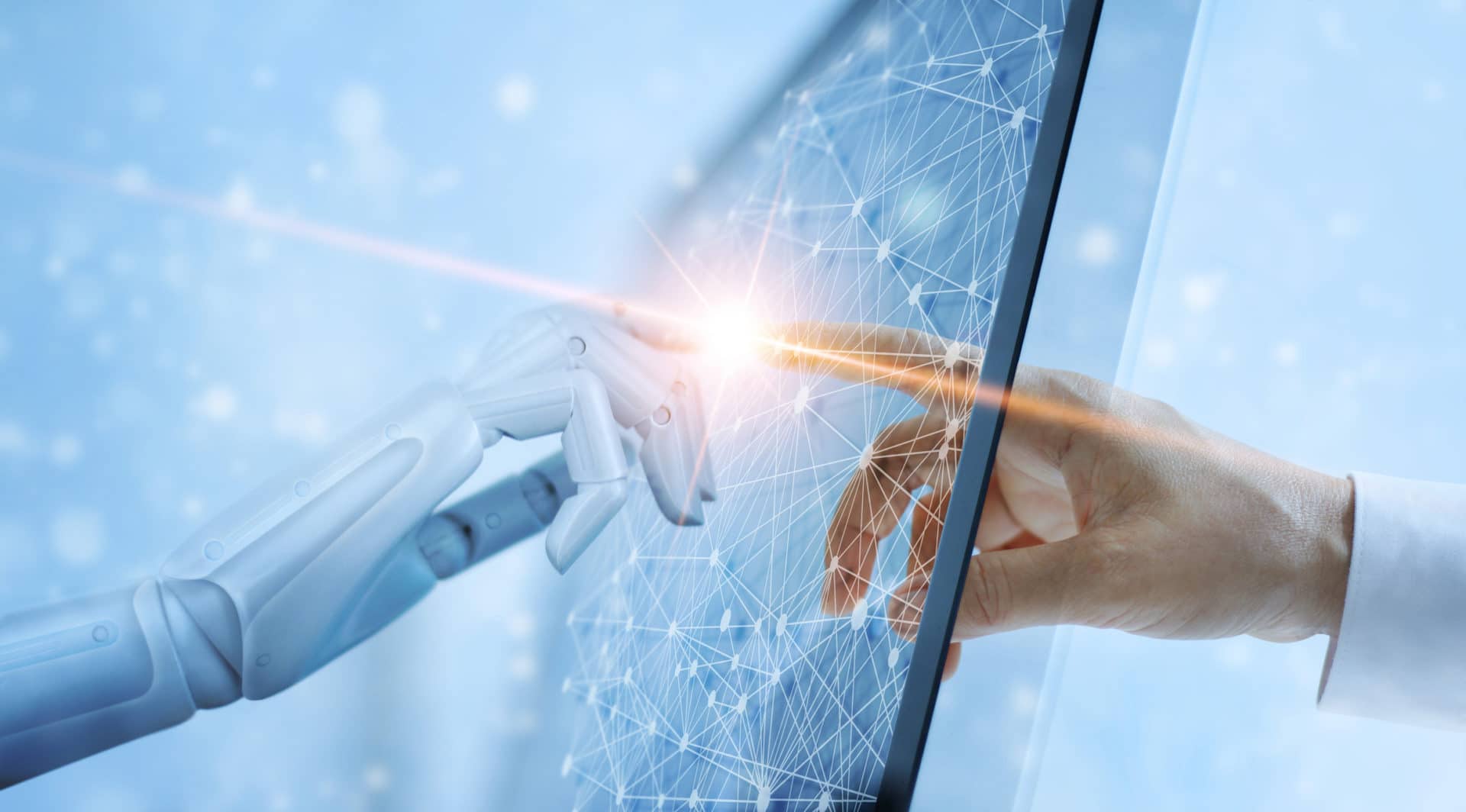
Leading-edge consumer technology companies, such as Google, Amazon, and Netflix, have demonstrated the impact that machine learning can have on the customer experience. These brands have become some of the most valuable in the world by delivering experiences that feel magical to the end consumer by using machine learning to make helpful recommendations, tag pictures, and translate documents. They’ve also made machine learning top of mind among executives at enterprises across all industries who recognize the need to adopt it to avoid being disrupted.
As consumers, we’re primarily aware of how machine learning impacts the ‘last mile’ aspects of the customer experience. But this technology is also readily applied to all areas of business operations. Data stewardship, an often ill-understood but vital part of DataOps, is one such area.
Data stewardship defined
As a data steward, you sit between raw data sources and data consumers, which include data scientists, data analysts, and business professionals. You are ultimately responsible for ensuring that data is well-managed and well-understood. This includes creating data dictionaries, monitoring and improving data quality, establishing governance, and defining the procedures required to meet security & privacy requirements.
Areas where machine learning can help
There is a range of applications for machine learning but at its core, it works great for pattern recognition. The best machine learning problems also have a clear outcome. You should not expect machine learning to answer questions that aren’t being asked, but you should expect it to identify patterns and provide insights that are not readily apparent. Sample applications of machine learning include classification, prediction, clustering, optimization, and anomaly detection.
Applying machine learning to data stewardship
The ratio between data consumers and data stewards is often significant. Large enterprises may have thousands of data consumers for every data steward. As a result, data stewards spend a significant amount of their time identifying patterns within data to determine how they should prioritize their time and what fixes they should implement.
This is what makes data stewardship such a ripe area for machine learning. The amount of data available to data stewards is significant, and it is impossible for them to keep up with the demands of their data consumer counterparts.
Five high impact ways that we’ve seen machine learning have a big impact on data stewardship include: identifying data sources, fixing data quality issues, mapping data sets to a schema, clustering similar records together, and classifying records. Our recommendation is to get started in one or two areas to gain comfort with the technology and deliver quick wins so that your organization will buy into adopting it more broadly.
Getting started: think small
You don’t need to boil the ocean to start seeing value from applying machine learning to data stewardship. Pick one domain (e.g., customer data) or one application (e.g., Salesforce) and start collecting feedback on its data. Tamr Steward, Jira, and Asana are all applications that we see being used broadly in this capacity. After hearing from consumers for 1 – 2 months, you will have more confidence in what problem to solve.
Getting a small, quick win is the key to being able to launch a broader machine learning initiative. Google, Amazon, and Netflix were experimenting with machine learning long before it became a pervasive part of the consumer experience. Transforming your data stewardship program into a differentiating force starts with gaining hands-on familiarity with how machine learning can make your data consumers more successful. The inherent scalability of the technology means that it won’t be long after realizing those quick wins that your data stewardship program becomes a competitive advantage.
Get a free, no-obligation 30-minute demo of Tamr.
Discover how our AI-native MDM solution can help you master your data with ease!