Avoiding the Dirty Data Dilemma in Snowflake with Tamr RealTime
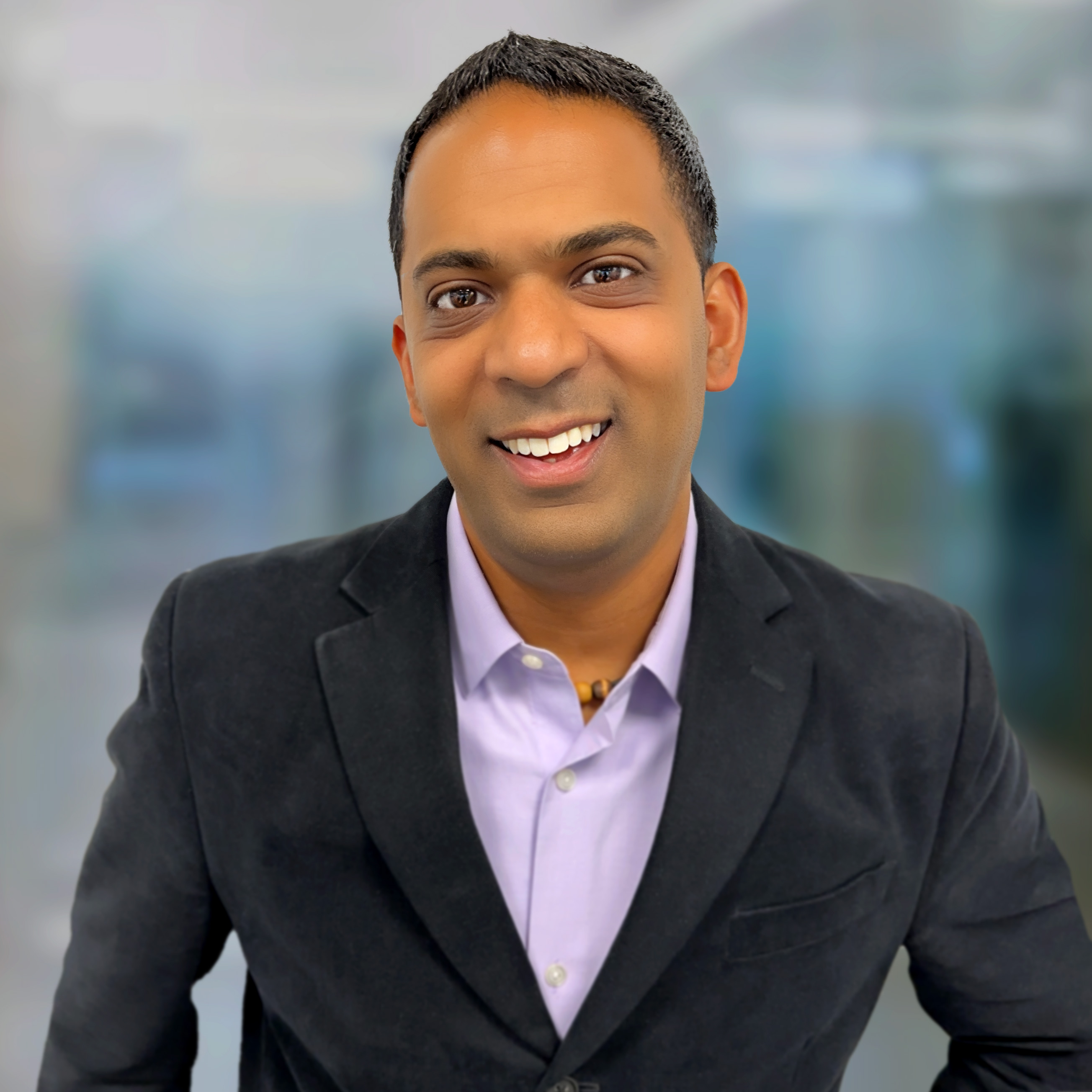

Summary:
- Migrating data to Snowflake is a major step in digital transformation, but poor data quality remains a challenge.
- Real-time processing and AI technology enable organizations to maintain clean, accurate golden records at scale.
- Tamr RealTime integrated with Snowflake helps proactively prevent duplicate data entry.
- Tamr RealTime allows for immediate identification and resolution of potential duplicates before they enter Snowflake.
- Investing in eliminating duplicate data now leads to improved data integrity, productivity, and decision-making in the future.
Migrating data to a cloud data warehouse like Snowflake represents a major step forward for organizations in their digital transformation journey. Not only do cloud data warehouses help to break down data silos, but they also promise to increase data accessibility and improve performance and scalability in order to deliver better, more reliable insights for business decision-making.
However, despite the myriad of benefits that cloud data warehouses provide, they are not a panacea that solves all of an organization's data-related challenges. One persistent issue that many organizations face, even after a successful migration of data into Snowflake, is poor data quality. Despite the robust infrastructure of cloud data warehouses, if the underlying data is inconsistent, incomplete, outdated, or inaccurate, the insights drawn from it can be misleading and potentially harmful to decision-making.
The Dirty Data Dilemma
Dirty data is an age-old issue. And it's costing organizations upwards of 12 million dollars each year. But despite efforts to clean and curate data using traditional master data management (MDM) tools, the problem persists. And to be quite frank, it's only getting worse.
Today’s proliferation of data sources is causing inconsistencies and inefficiencies to run rampant in organizations worldwide. And while many organizations have adopted data streaming architectures to keep information in sync across disparate systems, the addition of Snowflake as another system that holds data only exacerbates the problem. Humans become responsible for manually cleaning snapshots of data, and when they struggle to do so (which they inevitably will!), analyses quickly become out-of-date, inconsistent, and confusing, making it virtually impossible for users to identify accurate, trustworthy golden records.
Said differently, it's the adage "garbage in, garbage out" personified. Failing to clean data before it enters your new, pristine Snowflake environment is a significant misstep and one that could put the success of your migration to the cloud at risk.
Overcoming Data Quality Issues When Migrating to Snowflake
Preventing bad data from entering Snowflake is priority number one when it comes to a successful migration to the cloud. But too often, organizations decide to migrate all their existing data as is and then attempt to clean it up after the fact. In our experience, this strategy simply doesn't work. Because new data sources are emerging all the time, organizations that adopt this approach find it difficult, if not impossible, to keep up. As new records enter Snowflake, they are constantly playing catch-up, reacting to data quality issues as they arise instead of proactively resolving them at the point of data capture.
Case in point: customer onboarding. Customers expect that the organizations they work with have a holistic view of their relationship. But when duplicate customer records enter Snowflake, customer journeys become fragmented, causing customers to question if the organization really knows them at all. Having the ability to search for existing records before onboarding the new customer record is key to avoiding duplication and ensuring that all relevant information about the customer is captured in one trustworthy golden record.
Migrating unclean, duplicate data puts the successful adoption of Snowflake at risk, especially when data quality remains a manual, offline task. To overcome this challenge, organizations need the ability to clean data while it's in-motion - not just once it reaches its destination in Snowflake. By putting in place a mechanism that allows users to proactively search existing data and identify records that are a potential match, organizations can identify duplicate data at the point of entry and resolve the entities in real time, preventing the issue from the outset.
Eliminating Duplicate Data in Snowflake with Tamr RealTime
Tamr RealTime is a set of new features for Tamr's AI-native master data management platform that represent a significant leap forward in the ability to deliver clean, curated, and accurate data at scale. Tamr's AI-first approach enables data teams to adapt to changes quickly, By combining Tamr's real-time processing and the capabilities within Snowflake Streams, organizations can now clean data while it's still in-motion, enabling them to get the master data they need faster.
Through integration with Snowflake Streams, Tamr provides a better way to manage the change data capture process, making the vision of improving data in real-time a reality. Historically, the change data capture process was reactive. Organizations would wonder "what happened to my data" - but they would ask this question after the dirty data entered Snowflake. However, by integrating with Snowflake Streams, Tamr RealTime enables organizations to capture change and make improvements to the data proactively at the point of entry.
To illustrate this point, let's use supplier data as an example. Rather than adding a new supplier to Snowflake and realizing after-the-fact that multiple records for that supplier already exist, Tamr RealTime's search APIs enable organizations to find records that are either an exact or fuzzy match, providing immediate visibility into potential duplicates. Once identified, Tamr RealTime enables users to merge records so they can avoid adding duplicate records to Snowflake which preserves the overall integrity of the golden records.
Further, users can search for matching records using Tamr's persistent ID, TamrID, to locate records that are a match to the ones they wish to migrate into Snowflake. This real-time, ID-based lookup not only searches for current TamrIDs but also identifies historical IDs tied to the same records so that the search results return all possible matches across all data sets. This process, affectionately known as "swizzling," means that if a user searches for an outdated or unused ID, they will still find the best, most recent version of the record, even if the ID changed.
Tamr's approach flips the script: instead of loading data and reactively fixing it, Tamr RealTime allows organizations to proactively stop duplicate data from entering Snowflake in the first place. And once the new data enters the system, it is immediately available in search, with virtually no lag time at all.
The Benefits of Tamr RealTime and Snowflake Integration
Using Tamr RealTime integrated with Snowflake gives organizations the ability to democratize access to consistent data for everyone who needs it. Because Tamr RealTime is built on the ability to have a guaranteed persistent ID across the enterprise, every time someone queries that TamrID, they all receive the same, trustworthy golden record that represents the latest and best version of the data, based on the latest, real-time update.
Further, using Tamr RealTime, organizations can reveal duplicate records, even when they exist in disparate systems and sources before they enter Snowflake. By spotting duplicates up front, organizations can avoid adding to the data clutter, ensuring that the data within Snowflake remains integrated and pristine.
Finally, Tamr's AI-native data mastering solutions take full advantage of advanced AI to fix bad data at scale, while still incorporating the feedback and governance only humans can provide. When someone onboards potentially bad data in Snowflake, such as a new entity that looks similar to an existing one, Tamr flags that data in real-time so that a person can review it, creating a human-guided feedback loop that catches potential problems early. This powerful blend of AI and human refinement helps to ensure that the data remains clean and trustworthy, even as new sources emerge.
Eliminating duplicate data is a vital step in ensuring the accuracy and reliability of data entering the Snowflake environment. By proactively addressing duplicates, organizations not only streamline operations but also enhance the quality of insights that drive decision-making.
As organizations continue to rely on data as a critical asset, maintaining clean and deduplicated data in Snowflake is essential for achieving long-term success. By investing the time and resources now to eliminate duplicate data, organizations will reap the benefits of improved data integrity, increased productivity, and more confident decision-making in the future.
To discover how Tamr RealTime can help your organization can ensure the successful onboarding of clean data to your cloud data warehouse or agile data lakes, please request a demo.
Get a free, no-obligation 30-minute demo of Tamr.
Discover how our AI-native MDM solution can help you master your data with ease!